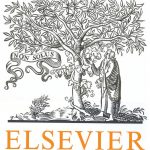
ترجمه مقاله نقش ضروری ارتباطات 6G با چشم انداز صنعت 4.0
- مبلغ: ۸۶,۰۰۰ تومان
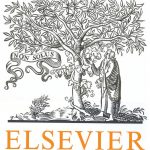
ترجمه مقاله پایداری توسعه شهری، تعدیل ساختار صنعتی و کارایی کاربری زمین
- مبلغ: ۹۱,۰۰۰ تومان
Abstract
Curse of dimensionality is a key issue in engineering optimization. When the dimension increases, distribution of samples becomes sparse due to expanded design space. To obtain accurate and reliable results, the amount of samples often grows exponentially with the dimensions. To improve the efficiency of the surrogate with limited samples, a Two-level Multi-surrogate Assisted Optimization (TMAO) is suggested. The framework of the TMAO is to decompose a complicated problem into separable and non-separable components. In the first-level, High Dimensional Model Representation (HDMR) is utilized to determine the correlations among input variables. Then, a high dimensional problem can be decomposed into separable and non-separable components. Thus, the dimension of the original problem might be reduced significantly. Moreover, considering noises and outliers, Support Vector Regression (SVR)-HDMR is utilized to obtain more reliable surrogate. Expected Improvement (EI) criterion is suggested to generate new samples to save computational cost. In the second-level, to handle the non-separable component, a multi-surrogate assisted sampling strategy is suggested. Compared with other methods, the distinctive characteristic of the suggested sampling strategy is to use different surrogates to search potential uncertain regions. Considering the diversity of surrogates, more feature samples might be generated close to the local optimum. Even though it is still difficult to find a global solution, it could help us to find a feasible solution in practice. To verify the performance of the suggested method, several high dimensional mathematical functions are tested by the suggested method. The results demonstrate that all test functions can be successfully solved.
6. Conclusions
In this study, an alternative SAO method called TMAO is suggested. The major characteristics of the TMAO are summarized as follows. TMAO is composed of two layers. In the first-level, the cut-HDMR modeling framework is used to decompose a high dimensional problem into combinations of low or medium dimensional ones. Compared with popular cut-HDMR, the SVR-HDMR is suggested to obtain more reliable surrogate. The test results also show that the SVR-HDMR achieves the best accurate model with noises and outliers compared with other cut-HDMRs. Moreover, to improve the efficiency of modeling, the EI criterion is also integrated. In the second-level, the GMDH framework is used to optimize the correlative input variables. Because some independent or weak correlative input variables might be filtered in the first-level, the GMDH is suitable. Furthermore, to enhance the robustness of modeling without adding more samples, the MAS is suggested. Compared with the MSEGO, the diversity of the sampling distribution can be promised by the LOOCV. In order to verify the performance of the TMAO, three tests are performed. The first one is to compare the performance of modeling approaches and sampling strategies. Based on this test, we select SVR-HDMR and EI sampling strategy as the best combination in the cut-HDMR framework. The second test is used to compare the performance ofthe TMAO and other recently developed algorithms, such as SAGA-GLS and MAES. Because we hope use the TMAO to apply to the real-world problems, the NFEs are limited to 1000. According to the results, the advantage of the TMAO is obvious. The final one is the test for the EGO, a well-known SAO method. Compared with the EGO, the efficiency of the TMAO is significantly improved. However, the TMAO has been investigated by several kinds of nonlinear functions. For some highly nonlinear problems (50d), the real global optimum is still difficult to be obtained in the limited NFEs. Moreover, the suggested TMAO is based on the assumption that the problem can be decomposed. Otherwise, only the secondlevel is available. Since it is difficult to determine the characteristic of the given problem before starting the TMAO, some samples might be wasted. We also hope to find a more efficient way to disclose the essential of the problem. Unfortunately, we still cannot apply the TMAO to a 50d problem yet because some geometrical parameters cannot be parametrized in practice and CAD and FE models cannot be integrated yet. In our opinion, this is another critical difficulty to prevent the application of the optimization algorithm.