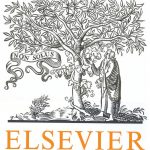
ترجمه مقاله نقش ضروری ارتباطات 6G با چشم انداز صنعت 4.0
- مبلغ: ۸۶,۰۰۰ تومان
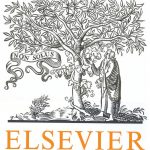
ترجمه مقاله پایداری توسعه شهری، تعدیل ساختار صنعتی و کارایی کاربری زمین
- مبلغ: ۹۱,۰۰۰ تومان
ABSTRACT
The prediction of next location for users in location-based social networks has become an increasing significant requirement since it can benefit both users and business. However, existing methods lack an integrated analysis of sequence context, input contexts and user preferences in a unified way, and result in an unsatisfactory prediction. Moreover, the interaction between different kinds of input contexts has not been investigated. In this paper, we propose a multi-context integrated deep neural network model (MCIDNN) to improve the accuracy of the next location prediction. In this model, we integrate sequence context, input contexts and user preferences into a cohesive framework. Firstly, we model sequence context and interaction of different kinds of input contexts jointly by extending the recurrent neural network to capture the semantic pattern of user behaviors from check-in records. After that, we design a feedforward neural network to capture high-level user preferences from check-in data and incorporate that into MCI-DNN. To deal with different kinds of input contexts in the form of multi-field categorical, we adopt embedding representation technology to automatically learn dense feature representations of input contexts. Experimental results on two typical real-world datasets show that the proposed model outperforms the current state-of-the-art approaches by about 57.12% for Foursquare and 76.4% for Gowalla on average regarding F1- score@5.
CONCLUSION
We have presented an integrated analysis of the joint effect of multiple factors, i.e., sequence context, input contexts, and user preferences, on the process of a user's decision to the next location. We have also developed an effective Multi-Context Integrated Deep Neural Network Model (MCI-DNN) to improve the accuracy of next location prediction. The prediction results by two datasets from Foursquare and Gowalla demonstrate the significant joint influence of sequence context and the interaction of different kinds of input contexts on the user's decision to the next locations. The average improvement in term of F1-score@5 was about 57.12% for Foursquare and 76.4% for Gowalla. The model developed herein performed better than the state-of-the-art approaches in view of prediction accuracy and stability. The proposed method shows significant potential for next location predictions in several applications where sequence context and input context characteristics exist, such as a recommendation system, advertising delivery, traffic jams forecasting, urban planning and so on.