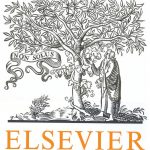
ترجمه مقاله نقش ضروری ارتباطات 6G با چشم انداز صنعت 4.0
- مبلغ: ۸۶,۰۰۰ تومان
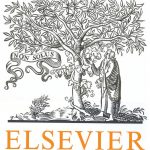
ترجمه مقاله پایداری توسعه شهری، تعدیل ساختار صنعتی و کارایی کاربری زمین
- مبلغ: ۹۱,۰۰۰ تومان
Abstract
Developing group recommender systems (GRSs) is a vital requirement in many online service systems to provide recommendations in contexts in which a group of users are involved. Unfortunately, GRSs cannot be effectively supported using traditional individual recommendation techniques because it needs new models to reach an agreement to satisfy all the members of this group, given their conflicting preferences. Our goal is to generate recommendations by taking each group member's contribution into account through weighting members according to their degrees of importance. To achieve this goal, we first propose a member contribution score (MCS) model, which employs the separable non-negative matrix factorization technique on a group rating matrix, to analyze the degree of importance of each member. A Manhattan distance-based local average rating (MLA) model is then developed to refine predictions by addressing the fat tail problem. By integrating the MCS and MLA models, a member contribution-based group recommendation (MC-GR) approach is developed. Experiments show that our MC-GR approach achieves a significant improvement in the performance of group recommendations. Lastly, using the MC-GR approach, we develop a group recommender system called GroTo that can effectively recommend activities to web-based tourist groups.
6. Conclusion and further study
In this paper, we have proposed a new group recommendation approach for modeling group profiles by considering all member contributions to the group's activities. We have also proposed a MCS model to measure the contribution of each group member in which, by partitioning the item space, we can analyze members' opinions using the SNMF technique. In addition, the MLA model has been proposed to alleviate the fat tail problem by adaptively calculating the average rating related to the target item when predicting unknown group ratings. Using these two models, we can handle a high level of compromise in the group profile and exclude unnecessary information when generating predictions of user preferences. The experiments were set up on two popular public datasets, and we have compared our approach with three popular approaches in the field of group recommendation. The results show the high effectiveness of our MCS-MLA approach. This study not only has theoretical significance but also potentially has high practical application. Many online services, such as movie or tourism recommendation sites and other websites, could adopt our approach. Our future study will include the extension of the proposed approach to select representative samplings instead of random samplings when sub-space differences are taken into consideration. A possible future improvement is to mathematically define a function to describe the degree of contribution divergence, and to incorporate alternative models when the function has a higher value.