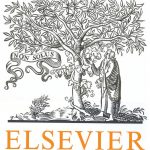
ترجمه مقاله نقش ضروری ارتباطات 6G با چشم انداز صنعت 4.0
- مبلغ: ۸۶,۰۰۰ تومان
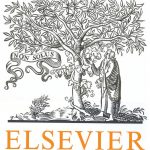
ترجمه مقاله پایداری توسعه شهری، تعدیل ساختار صنعتی و کارایی کاربری زمین
- مبلغ: ۹۱,۰۰۰ تومان
Abstract
Epilepsy is a chronic neurological condition that affects approximately 70 million people worldwide. Characterised by sudden bursts of excess electricity in the brain, manifesting as seizures, epilepsy is still not well understood when compared with other neurological disorders. Seizures often happen unexpectedly and attempting to predict them has been a research topic for the last 30 years. Electroencephalograms have been integral to these studies, as the recordings that they produce can capture the brain’s electrical signals. The diagnosis of epilepsy is usually made by a neurologist, but can be difficult to make in the early stages. Supporting para-clinical evidence obtained from magnetic resonance imaging and electroencephalography may enable clinicians to make a diagnosis of epilepsy and instigate treatment earlier. However, electroencephalogram capture and interpretation is time consuming and can be expensive due to the need for trained specialists to perform the interpretation. Automated detection of correlates of seizure activity generalised across different regions of the brain and across multiple subjects may be a solution. This paper explores this idea further and presents a supervised machine learning approach that classifies seizure and non-seizure records using an open dataset containing 342 records (171 seizures and 171 non-seizures). Our approach posits a new method for generalising seizure detection across different subjects without prior knowledge about the focal point of seizures. Our results show an improvement on existing studies with 88% for sensitivity, 88% for specificity and 93% for the area under the curve, with a 12% global error, using the k-NN classifier.
7. Conclusions and future work
Within a supervised-learning paradigm, this study has addressed this challenge by utilising EEG signals to classify seizure and non-seizure records. Our approach posits a new method for generalising seizure detection across different subjects without prior knowledge about the focal point of seizures. Our results show an improvement on existing studies with 88% for sensitivity, 88% for specificity and 93% for the area under the curve, with a 12% global error, using the kNN classifier. The results suggest that the algorithms in-situ with existing clinical systems and practices may enable clinicians to make a diagnosis of epilepsy and instigate treatment earlier. It can help to reduce costs by limiting the number of trained specialists required to perform the interpretation by automating the detection of correlates of seizure activity generalised across different regions of the brain and across multiple subjects. There are a large number of features reported in the literature, which have not been considered in this paper. In particular our future work will consider the set of features described in Logesparan et al. [34], Logesparan et al. [35]. Furthermore, our future work will investigate the use of more advanced machine learning algorithms, despite the good performance of the classifiers considered in this paper. In particular, we will investigate the use of convolutional neural networks [53] and SVM with different kernels [54].