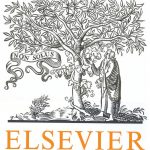
ترجمه مقاله نقش ضروری ارتباطات 6G با چشم انداز صنعت 4.0
- مبلغ: ۸۶,۰۰۰ تومان
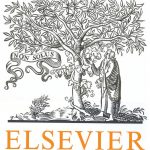
ترجمه مقاله پایداری توسعه شهری، تعدیل ساختار صنعتی و کارایی کاربری زمین
- مبلغ: ۹۱,۰۰۰ تومان
Abstract
To provide a scalable and flexible tool for 3D shape classification, this paper proposes an iterative 3D shape classification method by integrating incrementally updating, online learning and user intervention. It classifies the collection of 3D shapes iteratively by combing unsupervised clustering with online metric learning, and puts the user intervention into the loop of each iteration. The features of our method lie in three aspects. Firstly, it discovers the potential groups in the collection group by group without any pre-labeled samples or any pre-trained classifiers. Secondly, the users can get the desirable classes by directly confirming the required members of each group and annotate them with any free label to suit for different applications. Finally, the scalable collection can be handled dynamically and efficiently by our incrementally updating mechanism based on the online metric learning. The experimental results prove the effectiveness of the proposed method.
7. Conclusion
In this paper, we propose an iterative 3D shape classification method using online metric learning. The features of our method lie in three aspects. Firstly, the collection of 3D shapes can be classified group by group and iteratively. The unsupervised clustering, online metric learning and user intervention are integrated into a framework and work as a cohesive whole. Secondly, the users can classify the 3D shape collection flexibly and freely. They can get the desirable shape classifi- cation result without the prior determination about the scope and number of the categories. Finally, the scalable collection can be handled dynamically and efficiently. By our updating mechanism, our method can process the large scaled data set, and the dynamic increasing data set. Experimental results show that the proposed method improves both the effectiveness and efficiency of 3D shape classification.