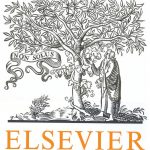
ترجمه مقاله نقش ضروری ارتباطات 6G با چشم انداز صنعت 4.0
- مبلغ: ۸۶,۰۰۰ تومان
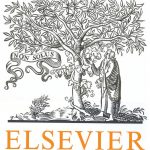
ترجمه مقاله پایداری توسعه شهری، تعدیل ساختار صنعتی و کارایی کاربری زمین
- مبلغ: ۹۱,۰۰۰ تومان
Abstract
Nearly all recent face recognition algorithms have been evaluated on the Labeled Faces in the Wild (LFW) dataset and many of them achieved over 99% accuracy. However, the performance is still not enough for real-world applications. One problem is the data bias. The faces in LFW and other web-collected datasets come from celebrities. They are quite different from the faces of a normal person captured in the daily life. In other words, they are different in the face distribution. Replacing the training data with the right distribution is a simple solution. However, the photos of common people are much harder to collect because of the privacy concerns. So it is useful to develop a method that transfers the knowledge in the data of different face distribution to help improving the final performance. In this paper, we crawl a large face dataset whose distribution is different from LFW and show the improvement of LFW accuracy with a simple domain adaptation technique. To the best of our knowledge, it is the first time that domain adaptation is applied in the unconstrained face recognition problem with million scale dataset. Besides, we incorporate face verification threshold into FaceNet triplet loss function explicitly. Finally, we achieve 99.33% on the LFW benchmark with only single CNN model and similar performance even without face alignment.
Conclusion
In this paper, we crawl a million scale face dataset called TaoMM whose distribution is different from LFW. By employing a simple domain adaptation technique, we improve the LFW accuracy even with a million scale target domain dataset. By incorporating face verification threshold θ into FaceNet triplet loss explicitly, we reduce the LFW error rate by 26.9%. Finally, We achieve 99.33% on the LFW benchmark with only single CNN model and similar performance even without face alignment by applying aggressive data augmentation. When compared without face alignment, we achieve 99.28% which is better than FaceNet 98.87%, even if FaceNet uses a much larger dataset with 200M images, about 44 times of ours. Further work will focus on applying more complex domain adaptation technique to fully exploit the knowledge in the source domain to help improving the performance of target domain. We will also look into the effect of large λ in Eq. (2) when we pursue high True Accept Rate at extreme low False Accept Rate in face verification.