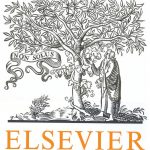
ترجمه مقاله نقش ضروری ارتباطات 6G با چشم انداز صنعت 4.0
- مبلغ: ۸۶,۰۰۰ تومان
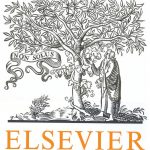
ترجمه مقاله پایداری توسعه شهری، تعدیل ساختار صنعتی و کارایی کاربری زمین
- مبلغ: ۹۱,۰۰۰ تومان
abstract
Data mining and machine learning approaches can be used to predict breast cancer recurrence. However, real datasets often include missing values for various reasons. In this paper, a hybrid imputation method is proposed with respect to the dependency between the attributes and the type of incomplete attributes in order to especially improve the prediction of breast cancer recurrence. After splitting the dataset into two discrete and numerical subsets, first missing values of the discrete fields are imputed using Bayesian network. Then, using Tensor factorization, the integrated dataset, which comprises of the filled-subset of the previous stage and numerical missing values subset, is constructed so that both continuous missing values are imputed and the accuracy of imputation is enhanced. We evaluated the proposed method versus six imputation methods i.e. mean, Hot-deck, K-NN, Weighted K-NN, Tensor factorization and Bayesian network on three datasets and used three classifiers, namely decision tree, K-Nearest Neighbor and Support Vector Machine for recurrence prediction. Experimental results show that the proposed method has as average 0.26 prediction improvement. Also, the prediction performance of the proposed approach outperforms all other imputation-classifier pairs in terms of specificity, sensitivity and accuracy.
6. Discussion and conclusion
The recurrence of breast cancer affects the lives of patients even many years after Decision TreeKNNSVMsurgery. In recent years, machine learning and data mining methods have increasingly improved predictions and helped medical professionals. Extracting verified information from collected medical data is considered as a major challenge. Due to the increase of cancer patients, especially breast cancer patients, the research about this field is very important. The existence of missing values in medical data is a main challenge in this field. A precise estimation of missing values, which leads to better decision, or at least assist experts for this purpose, is valuable in cancer diagnosis and recurrence prediction. In this paper, a new approach for missing values imputation is proposed with respect to dependencies among variables and the type of incomplete variable which significantly affects imputation using Tensor and Bayesian networks for both categorical and numerical variables. The proposed method for replacing missing data has also been evaluated using several different imputation methods including mean, Hot-deck, K-NN, Weighted K-NN, Tensor and Bayesian network using NRMSE criterion. Although both tensor and Bayesian networks are capable of imputing missing values, they are more capable in continuous and discrete missing data imputation, respectively. We also used three predictive models, namely, SVM, K-NN and DT and three popular measures of accuracy, sensitivity and specificity in order to predict breast cancer recurrence. These measures are applied using 5-fold cross validation on all datasets created by imputation methods. Finally, experimental results are reported for each imputation-classifier pair.