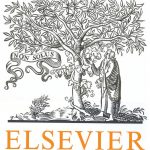
ترجمه مقاله نقش ضروری ارتباطات 6G با چشم انداز صنعت 4.0
- مبلغ: ۸۶,۰۰۰ تومان
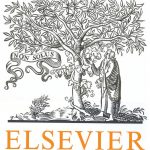
ترجمه مقاله پایداری توسعه شهری، تعدیل ساختار صنعتی و کارایی کاربری زمین
- مبلغ: ۹۱,۰۰۰ تومان
bstract
A simple and novel approach to identify the clusters based on structural and attribute similarity in graph network is proposed which is a fundamental task in community detection. We identify the dense nodes using Local Outlier Factor (LOF) approach that measures the degree of outlierness, forms a basic intuition for generating the initial core nodes for the clusters. Structural Similarity is identified using k-neighbourhood and Attribute similarity is estimated through Similarity Score among the nodes in the group of structural clusters. An objective function is defined to have quick convergence in the proposed algorithm. Through extensive experiments on dataset (DBLP) with varying sizes, we demonstrate the effectiveness and efficiency of our proposed algorithm k-Neighbourhood Attribute Structural (kNAS) over state-of-the-art methods which attempt to partition the graph based on structural and attribute similarity in field of community detection. Additionally, we find the qualitative and quantitative benefit of combining both the similarities in graph.
6. Conclusion
In this paper, we have proposed a new approach (kNAS)for overlapping community detection in large scale graph by combining the topological and attribute similarity. The large graph is partitioned into m clusters having high intracluster structural similarity and low intercluster similarity. The initial centroids of the cluster are automatically selected based on Local Outlier Factor instead of random selection of centers. Iteratively the centroids updated to the vertex closest to average density of the cluster. The vertices are assigned to cluster that are within the k-distance and have similar attributes with the centroid. The structural similarity is based on k-neighbourhood vertex and attribute similarity is based on the Similarity Score. Moreover,two evaluation measures are defined to measure the quality of the cluster formed by four algorithms. Our experiment demonstrates that kNAS algorithm outperforms stateof-art methods in quality and efficiency with respect to varied size datasets.