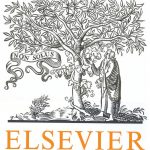
ترجمه مقاله نقش ضروری ارتباطات 6G با چشم انداز صنعت 4.0
- مبلغ: ۸۶,۰۰۰ تومان
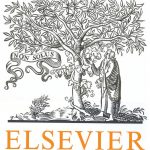
ترجمه مقاله پایداری توسعه شهری، تعدیل ساختار صنعتی و کارایی کاربری زمین
- مبلغ: ۹۱,۰۰۰ تومان
Abstract
This paper is about authenticating genuine van Gogh paintings from forgeries. The paintings used in the test in this paper are provided by van Gogh Museum and Kröller-Müller Museum. The authentication process depends on two key steps: feature extraction and outlier detection. In this paper, a geometric tight frame and some simple statistics of the tight frame coefficients are used to extract features from the paintings. Then a forward stage-wise rank boosting is used to select a small set of features for more accurate classification so that van Gogh paintings are highly concentrated towards some center point while forgeries are spread out as outliers. Numerical results show that our method can achieve 86.08% classification accuracy under the leave-one-out cross-validation procedure. Our method also identifies five features that are much more predominant than other features. Using just these five features for classification, our method can give 88.61% classification accuracy which is the highest so far reported in literature. Evaluation of the five features is also performed on two hundred datasets generated by bootstrap sampling with replacement. The median and the mean are 88.61% and 87.77% respectively. Our results show that a small set of statistics of the tight frame coefficients along certain orientations can serve as discriminative features for van Gogh paintings. It is more important to look at the tail distributions of such directional coefficients than mean values and standard deviations. It reflects a highly consistent style in van Gogh's brushstroke movements, where many forgeries demonstrate a more diverse spread in these features.
7. Conclusion
We have proposed a geometric tight frame based visual stylometry method to discriminate paintings by van Gogh from those by imitators. The methodology consists of some simple statistics of the geometric tight frame coefficients, as well as a boosting procedure for feature selection. Our methodology has been tested on a data set of 79 paintings provided by the van Gogh museum and Kröller-Muller museum. The classification accuracy of our method is 86.08%. The high classification accuracy shows that our features are appropriate in identifying the authorship of van Gogh’s paintings. In particular, our method identifies five robust features such that van Gogh’s paintings show a higher degree of similarity in that feature space while forgeries exhibit a wider spread tendency as outliers. The accuracy using these 5 features is 88.61% which is the best one compared with the existing methods so far (see Tables 2 and 4). The success of this small set of features reflects the consistency of van Gogh’s habitual brushstroke movements. From our results, we see that the “statistical outliers” of certain tight frame coefficients are not noise, but important signals to distinguish van Gogh’s painting from his contemporaries. Such “outliers” and their tail distributions may due to the intrinsic creativity of the maestro expressed through his brushstroke styles. We hope these features may help art scholars to find new digital evidences in van Gogh’s art authentication. In this paper, we did not discuss how the digitization or quantization may affect our results. We expect higher order filters are more sensitive to small changes in pixel values resulting from digitization or quantization. In our future study, we will consider how robust these filters are with respect to these or other possible errors. Our methodology can easily be generalized to authenticate paintings for other artists and that will also be our future research directions.