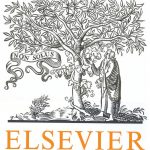
ترجمه مقاله نقش ضروری ارتباطات 6G با چشم انداز صنعت 4.0
- مبلغ: ۸۶,۰۰۰ تومان
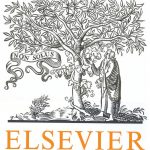
ترجمه مقاله پایداری توسعه شهری، تعدیل ساختار صنعتی و کارایی کاربری زمین
- مبلغ: ۹۱,۰۰۰ تومان
abstract
This work deals with the characterization of a hyperelastic material and the subsequent validation in different stressed states. The well-known three-parameter Mooney–Rivlin model is chosen for the sake of simplicity. In order to obtain the mechanical properties of this material, a specimen is tested using tensile forces. Once the tests are performed, the material constants are determined using a genetic algorithm to fit the experimental curve. An accurate fitness function is defined and the procedure to obtain the generations is described. Finally, with the aim to model and validate the results in a more complex setting, physical tests are performed in combination with numerical studies and FEM simulations.
6. Conclusion
This study shows the possibility to use genetic algorithms to fit experimental data, in order to obtain the necessary parameters to model hyperelastic materials in a FE software. During the study, a functional genetic algorithm was designed to fit Mooney–Rivlin parameters according to real data. This kind of algorithms has the main advantage that no differentiation is needed in order to establish a searching direction as to Newton methods, and so the fitness function could be non-differentiable. In addition, the use of genetic algorithms allows us to include in a very simple way test data with noise and even to extend the algorithm to use data from several tests (uniaxial, biaxial, shear) if available. Indeed, it is possible to think about the possibility to perform multi-objective optimization and to characterize materials with more complex behaviour including effects as nonlinear viscoelasticity or creep.
Finally, once the curve fitting is solved several times by the algorithm, three possible candidates are computed. The difference between these models is negligible in the domain of the test data but, far from this domain, the three models begin to diverge. The three candidates are also tested in a FE model using a more complex load case. In view of the results obtained using this FE model, the same conclusion could be taken. As a final conclusion, it is important to point out that the genetic algorithm is able to fit a Mooney–Rivlin model and to generate useful parameters which could be included into a FE model, but the test data used to compute the material parameters must be obtained from the strain level where the real component is considered.