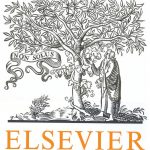
ترجمه مقاله نقش ضروری ارتباطات 6G با چشم انداز صنعت 4.0
- مبلغ: ۸۶,۰۰۰ تومان
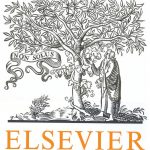
ترجمه مقاله پایداری توسعه شهری، تعدیل ساختار صنعتی و کارایی کاربری زمین
- مبلغ: ۹۱,۰۰۰ تومان
Abstract
Resource allocation in multi-cloud computing is a complicated chore; there are many constraints and configuration in accordance with cloud providers as well as cloud customers. Mapping the incoming job request to available virtual machines (VMs) is a non-polynomial complete problem as the nature of traffic quite arbitrary. Customer requirements and capacity of applications change frequently. To bridge the gap between frequently changing customer requirement and available infrastructure for the services, we propose Genetic Algorithm-based Customer-Conscious Resource Allocation and Task Scheduling in multi-cloud computing. The algorithm is basically divided into two phases, namely genetic algorithm-based resource allocation and shortest task first scheduling. The objective is to map the tasks to VMs of the multi-cloud federation in order to have minimum makespan time and maximum customer satisfaction. Rigorous experiments were done on synthetic data and compared the simulation results with the existing scheduling algorithm. Results of simulation illustrate that the proposed algorithm outrun the existing ones as per concerned metrics.
6 Conclusion
To accommodate sporadic workload in the multi-cloud environment, where the capacity of job request may change dynamically, machine learning provides better resource allocation and task scheduling. A novel task scheduling algorithm termed as Genetic Algorithm-based CustomerConscious Resource Allocation and Task Scheduling (GACCRATS) is proposed for the heterogeneous multi-cloud environment. We compared the algorithm performance of COTS, TLBO and GACCRATS. COTS aims to maximise the customer satisfaction and surplus customer expectation, TLBO is used to find the task-VM pair having minimum makespan time, whereas GACCRATS objective is the minimisation of makespan time of tasks and maximisation of customer satisfaction rate. The algorithm mainly has two phases, mapping and scheduling the mapped tasks. Multiple instances are carried out with different compositions of datasets in MATLAB. The results show that the performance of the proposed algorithm has outperformed existing algorithms. Scalability of the simulated multi-cloud environment is considerably high. Data locality cost, latency arbitration, energy consumption and running cost of multi-cloud environment are out of the scope of the simulated scenario.