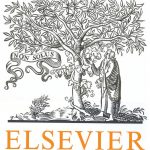
ترجمه مقاله نقش ضروری ارتباطات 6G با چشم انداز صنعت 4.0
- مبلغ: ۸۶,۰۰۰ تومان
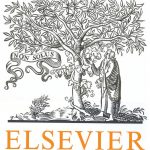
ترجمه مقاله پایداری توسعه شهری، تعدیل ساختار صنعتی و کارایی کاربری زمین
- مبلغ: ۹۱,۰۰۰ تومان
abstract
A framework for the forecasting of composite time series, such as market shares, is proposed. Based on Gaussian multi-series innovations state space models, it relies on the log-ratio function to transform the observed shares (proportions) onto the real line. The models possess an unrestricted covariance matrix, but also have certain structural elements that are common to all series, which is proved to be both necessary and sufficient to ensure that the predictions of shares are invariant to the choice of base series. The framework includes a computationally efficient maximum likelihood approach to estimation, relying on exponential smoothing methods, which can be adapted to handle series that start late or finish early (new or withdrawn products). Simulated joint prediction distributions provide approximations to the required prediction distributions of individual shares and the associated quantities of interest. The approach is illustrated on US automobile market share data for the period 1961–2013. © 2017 International Institute of Forecasters. Published by Elsevier B.V. All rights reserved.
7. Discussion
This paper has developed a state space approach for the forecasting of compositional time series that is invariant to the choice of the base series in the log-ratio transformation and satisfies the constraints that the predicted proportions must be non-negative and sum to one. The associated models may be extended to include seasonal patterns and explanatory variables, provided that common parameters are specified for the state variables, as is the case in the basic models defined in Table 1. The coefficients for the explanatory variables will be different in each equation, as those inputs have differential effects on shares (e.g., falling oil prices boost the sales of SUVs). For non-seasonal schemes in particular, the notion of local momentum may be useful for describing changes in shares, and it also requires one fewer parameter, which could be important when only short series are available.