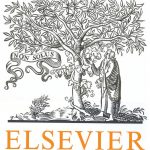
ترجمه مقاله نقش ضروری ارتباطات 6G با چشم انداز صنعت 4.0
- مبلغ: ۸۶,۰۰۰ تومان
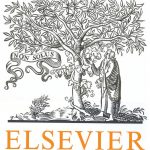
ترجمه مقاله پایداری توسعه شهری، تعدیل ساختار صنعتی و کارایی کاربری زمین
- مبلغ: ۹۱,۰۰۰ تومان
Abstract
Most of the opportunistic scheduling algorithms in literature assume that full wireless channel state information (CSI) is available for the scheduler. However, in practice obtaining full CSI may introduce a significant overhead. In this paper, we present a learning-based scheduling algorithm which operates with partial CSI under general wireless channel conditions. The proposed algorithm predicts the instantaneous channel rates by employing a Bayesian approach and using Gaussian process regression. It quantifies the uncertainty in the predictions by adopting an entropy measure from information theory and integrates the uncertainty to the decision-making process. It is analytically proven that the proposed algorithm achieves an ϵ fraction of the full rate region that can be achieved only when full CSI is available. Numerical analysis conducted for a CDMA based cellular network operating with high data rate (HDR) protocol, demonstrate that the full rate region can be achieved our proposed algorithm by probing less than 50% of all user channels.
7. Conclusion
In this paper, we considered scheduling in a downlink multiuser setting, where the base station can only probe a limited number of users due to the limited bandwidth on the uplink feedback channel. We have presented a joint scheduling and channel probing algorithm that can operate in a stationary and non-stationary network scenarios. The algorithm is based on an active learning framework that quantifies the reward of learning the current state of the system by using the entropy measure. Based on this measure, the scheduler makes an intelligent trade off between having a more up-to-date picture of the system and maximizing the overall system throughput. The proposed algorithm first decides the set of channels that should be probed at the beginning of each time slot. The set of channels is determined by considering not only the queue sizes and the estimated transmission rates but also the information to be obtained by probing a channel. We apply Gaussian Process Regression technique to predict CSI at each time slot based on the previously observed CSI. In numerical results, we show that the base station using MOSF can stabilize the network and achieve a similar delay performance as compared to full CSI Max-Weight algorithm by probing less than half of the users at every slot. Possible directions for future work include the investigation of the case where the channel gain changes within a time slot. Another possible future direction is to investigate the scheduling problem with incomplete CSI when interference from neighboring cells is present.