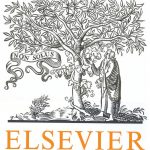
ترجمه مقاله نقش ضروری ارتباطات 6G با چشم انداز صنعت 4.0
- مبلغ: ۸۶,۰۰۰ تومان
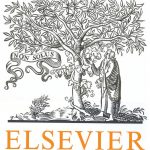
ترجمه مقاله پایداری توسعه شهری، تعدیل ساختار صنعتی و کارایی کاربری زمین
- مبلغ: ۹۱,۰۰۰ تومان
abstract
Endogeneity bias represents a critical issue for the analysis of cause and effect relationships. Although the existence of endogeneity can produce severely biased results, it has hitherto received only limited attention from researchers in marketing and related disciplines. Thus, this article aims to sensitize researchers intending to publish in the Industrial Marketing Management (IMM) journal to the topic of endogeneity. It outlines the problem of endogeneity bias, and provides an overview of potential sources, i.e. omission of variables, errors-in-variables, and simultaneous causality. Furthermore, the article shows ways to deal with endogeneity, including techniques based on instrumental variables as well as instrument-free approaches. Our methodological contribution relates to providing researchers aiming to publish in IMM with an initial overview of the causes of and remedies for endogeneity bias, which should be considered in designing research projects as well as when analysing data to obtain insights into cause and effect relationships (causal models)
4. Conclusions
Research has demonstrated what could happen when no actions are taken to correct for endogeneity (Villas-Boas & Winer, 1999). The outcomes clearly show that not accounting for endogeneity can result in misleading results, incorrect effects and inflated estimate levels in the model in comparison with analyses achieved when endogeneity corrections took place. Thus, if the researcher suspects the presence of endogeneity, the first logical step would be to identify the source of it, in order to proceed with the most suitable treatment. In line with the approaches mentioned in the previous section of this article, it is important for researchers to clearly realize which methods they can and should use to address the specific problem of endogeneity, which they face in their research. While in some cases several techniques might be equally applicable and suitable to implement, the decision concerning endogeneity corrections should be based on several factors, such as research design and data collection instrument, sample size, complexity of the model, and underlying theory and research context. Additionally to the remedies discussed, researchers are also urged to consider alternative ways of dealing with endogeneity issues. First of all, the research community publishing in IMM ought to endeavor to collect better quality data. This could be achieved via collecting additional relevant data (surveys and experiments) that could help explain hypothesized effects (Liu, Otter, & Allenby, 2007; Swait & Andrews, 2003). Another solution could be to make explicit ex ante assumptions about the nature of the endogeneity (i.e. use a strong theory to enhance conceptual arguments) and directly incorporate that relationship into the estimation (e.g. Aaker & Bagozzi, 1979). Overall, analysis and correction for endogeneity bias ought to become standard practice for causal modeling in articles published in IMM, similar to how non-response bias, common method bias, and outer measurement model analyses regarding validity and reliability have become part of the standard quality assurances and reporting templates.