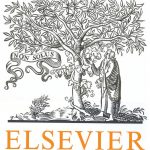
ترجمه مقاله نقش ضروری ارتباطات 6G با چشم انداز صنعت 4.0
- مبلغ: ۸۶,۰۰۰ تومان
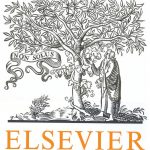
ترجمه مقاله پایداری توسعه شهری، تعدیل ساختار صنعتی و کارایی کاربری زمین
- مبلغ: ۹۱,۰۰۰ تومان
ABSTRACT
Artificial intelligence (AI) is proving to be an efficient alternative approach to classical modeling techniques. AI refers to the branch of computer science that develops machines and software with human-like intelligence. Compared to traditional methods, AI offers advantages to deal with problems associated with uncertainties and is an effective aid to solve such complex problems. In addition, AI-based solutions are good alternatives to determine engineering design parameters when testing is not possible, thus resulting in significant savings in terms of human time and effort spent in experiments. AI is also able to make the process of decision making faster, decrease error rates, and increase computational efficiency. Among the different AI techniques, machine learning (ML), pattern recognition (PR), and deep learning (DL) have recently acquired considerable attention and are establishing themselves as a new class of intelligent methods for use in structural engineering. The objective of this review paper is to summarize techniques concerning applications of the noted AI methods in structural engineering developed over the last decade. First, a general introduction to AI is presented and the importance of AI in structural engineering is described. Thereafter, a review of recent applications of ML, PR, and DL in the field is provided, and the capability of such methods to address the restrictions of conventional models are discussed. Further, the advantages of employing such algorithmic methods are discussed in detail. Finally, potential research avenues and emerging trends for employing ML, PR, and DL are presented, and their limitations are discussed.
Conclusions
This review paper presented the significance of emerging AI methods for structural engineering applications during the last decade. The survey indicated that among the numerous AI methods, pattern recognition (PR), machine learning (ML), and deep learning (DL) have been increasingly adapted and used for SHM and damage identification, optimization, modeling concrete properties, structural identification, earthquake engineering, etc. Yet, the common use of the noted methods has been for interpreting sensor data in SHM. The survey revealed that ML, PR, and DL algorithmic techniques have the ability to learn complicated interrelations among the contributing parameters, and thus allow solving a diversity of problems that are difficult, or not possible, to solve with traditional methods. Based on the literature survey, potential research avenues for employing PR, ML, and DL were also presented. Considering the emerging use of wireless sensor networks (e.g., self-powered sensor networks), ML- and PR-based models could become the next generation approaches to conduct non-destructive structural and material evaluation in SHM. This review showed that ML methods are able to discover hidden information about the structure’s performance by learning the influence of various damage or degrading mechanisms and the data collected from sensors, leading to reliable and efficient SHM frameworks. The literature further suggests that ML and DL techniques could also be applied to the computational mechanics domain, such as to optimize processes in the finite element method to enhance computational efficiency. These methods can also be used to solve complex problems through the novel concept of the Internet of Things (IoT).