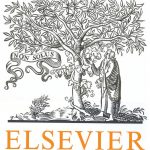
ترجمه مقاله نقش ضروری ارتباطات 6G با چشم انداز صنعت 4.0
- مبلغ: ۸۶,۰۰۰ تومان
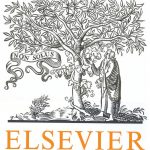
ترجمه مقاله پایداری توسعه شهری، تعدیل ساختار صنعتی و کارایی کاربری زمین
- مبلغ: ۹۱,۰۰۰ تومان
ABSTRACT
In this paper, we present a new automatic screening method to assess whether a patient from ambulatory care or emergency should be referred to a cardiology service. This method is based on deep neural networks with pretraining and takes as an input a raw ECG signal without annotation. This work is based on a prospective clinical study that took place at Hospital Clínic in Barcelona between 2011–2012 and recruited 1390 patients. For each patient, we recorded a 12-lead ECG and the diagnosis was conducted by the cardiology service at the same hospital. Normal, borderline normal and normal variant ECGs were labelled as normal and the rest as abnormal. Our deep neural networks with pretraining were tested through cross-validation with a cohort of 416 test patients. The performance of our model was compared against other standard classification methods such as neural networks without pretraining, Support Vector Machines, Extreme Learning Machines, kNearest Neighbours and a professional classification algorithm certified for medical use that annotates the raw ECG signals prior to classification. The resulting best classifier was a pretrained neural network with three hidden layers and 700 units in every layer. This network yielded an accuracy of 0.8552, a sensitivity of 0.9176 and a specificity of 0.7827. The best alternative classification method was a Support Vector Machine with a Gaussian kernel, which yielded an accuracy of 0.8476, a sensitivity of 0.9446 and a specificity of 0.7346. The professional classification algorithm yielded an accuracy of 0.8407, a sensitivity of 0.8558 and a specificity of 0.8214. Neural networks with pretraining automatically obtain a representation of the input data without resorting to any annotation and, thus, simplify the process of assessing normality of ECG signals. The results that we have obtained are slightly better than those obtained with the professional classification system and, for some network configurations, they can be considered as exchangeable. Neural networks with pretraining open up a promising line of research for the automatic assessment of ECG signals that may be used in the future in clinical practice.
4. Discussion
In this paper, neural networks with different architectures were trained with RBM-based pretraining and applied to a data set of 1390 pre-processed 12 lead ECGs. The deep networks presentedhere provide accurate and medically actionable results for ECG screening while keeping an acceptable balance between the different parameters of interest (accuracy, sensitivity and specificity). The generalization capabilities ofthe networks studied in this paper are acceptable and outperform other standard methods such as HES, neural networks without pretraining, SVMs, ELMs and kNNs. The pretraining procedure clearly plays an important role in improving the accuracy of the classifiers developed and helps to balance the rate between true and false positives. However, this improvementinaccuracy, sensitivity andspecificity comes at ahigh computational cost for training the deep neural networks.