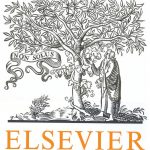
ترجمه مقاله نقش ضروری ارتباطات 6G با چشم انداز صنعت 4.0
- مبلغ: ۸۶,۰۰۰ تومان
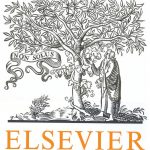
ترجمه مقاله پایداری توسعه شهری، تعدیل ساختار صنعتی و کارایی کاربری زمین
- مبلغ: ۹۱,۰۰۰ تومان
Abstract
Cloud computing is a type of parallel distributed computing system that has become a frequently used computer application. MapReduce is an effective programming model used in cloud computing and large-scale data-parallel applications. Hadoop is an open-source implementation of the MapReduce model, and is usually used for data-intensive applications such as data mining and web indexing. The current Hadoop implementation assumes that every node in a cluster has the same computing capacity and that the tasks are data-local, which may increase extra overhead and reduce MapReduce performance. This paper proposes a data placement algorithm to resolve the unbalanced node workload problem. The proposed method can dynamically adapt and balance data stored in each node based on the computing capacity of each node in a heterogeneous Hadoop cluster. The proposed method can reduce data transfer time to achieve improved Hadoop performance. The experimental results show that the dynamic data placement policy can decrease the time of execution and improve Hadoop performance in a heterogeneous cluster.
5. Conclusion
This paper proposes a data placement policy (DDP) for map tasks of data locality to allocate data blocks. The Hadoop default data placement strategy is assumed to be applied in a homogeneous environment. In a homogeneous cluster, the Hadoop strategy can make full use of the resources of each node. However, in a heterogeneous environment, a produces load imbalance creates the necessity to spend additional overhead. The proposed DDP algorithm is based on the different computing capacities of nodes to allocate data blocks, thereby improving data locality and reducing the additional overhead to enhance Hadoop performance. Finally in the experiment, for two types of applications, WordCount and Grep, the execution time of the DDP compared with the Hadoop default policy was improved. Regarding WordCount, the DDP can improve by up to 24.7%, with an average improvement of 14.5%. Regarding Grep, the DDP can improve by up to 32.1%, with an average improvement of 23.5%. In the future, we will focus on other types of jobs to improve Hadoop performance.