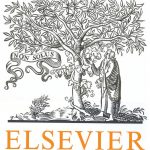
ترجمه مقاله نقش ضروری ارتباطات 6G با چشم انداز صنعت 4.0
- مبلغ: ۸۶,۰۰۰ تومان
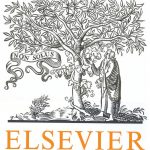
ترجمه مقاله پایداری توسعه شهری، تعدیل ساختار صنعتی و کارایی کاربری زمین
- مبلغ: ۹۱,۰۰۰ تومان
Abstract
With the development of information network, the popularity of Internet of Things (IoT) is an irreversible trend, and the intelligent demands for IoT is becoming more and more urgent. How to improve the cognitive ability of IoT is a new challenge and therefore has given rise to the emergence of Cognitive Internet of Things (CIoT). In this paper, a device level multimodal data correlation mining (DMDC) model is firstly designed based on the CCA to transform the data feature into a subspace and analyze the data correlation. The correlation of the device is obtained based on the comprehensive of data correlation and the location information of the device. Then a heterogeneous clustering model (HDC) is proposed by using the result of the correlation analysis to classify the device. Finally, we propose a device clustering algorithm based on multimodal data correlation (DCMDC) for CIoT, which combines the functions of multimodal data correlation analyze with device clustering. Extensive simulations are carried out and our results show that the proposed algorithm can effectively improve the quality of data transmission and the intelligent service.
CONCLUSION
To increase the data cognitive ability of CIoT, this paper introduces a device clustering algorithm based on multimodal data correlation which including the function of data correlation analyze and device clustering. A device-level multimodal data correlation mining model is firstly proposed based on the CCA algorithm to analyze the multimodal data and device correlation, which is capable of classifying the device according to the data correlation and device distribution. The DCMDC clusters the heterogeneous devices in CIoT according to their correlation by using the result of the data correlation mining model. Extensive simulations are performed to evaluate the proposed algorithm. The results show that the designed algorithm can achieve a satisfying quality of device clustering and has the potential to transform into a practical technique in CIoT.