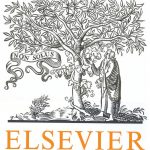
ترجمه مقاله نقش ضروری ارتباطات 6G با چشم انداز صنعت 4.0
- مبلغ: ۸۶,۰۰۰ تومان
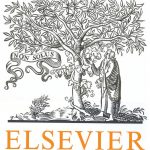
ترجمه مقاله پایداری توسعه شهری، تعدیل ساختار صنعتی و کارایی کاربری زمین
- مبلغ: ۹۱,۰۰۰ تومان
ABSTRACT
Excessive stress will lower work efficiency, lead to negative emotions and even various illnesses. This paper aims at detecting work-related stress based on physiological signals measured by a wearable device. Different from common binary stress detection, this study detects three levels of stress, i.e., no stress, moderate stress and high perceived stress. The Montreal Imaging Stress Task (MIST) is used to simulate the different stress conditions, including both mental stress and psychosocial stress factors that are relevant at the workplace. A sensor-based wearable device is used to acquire the electrocardiogram (ECG) and respiration (RSP) signals from 39 participants. We extract stress-related features from ECG and RSP, and the Random Forest is used to select the optimal feature combination, which is later fed to the classifier. Four classifiers are investigated about their ability to predict the three stress levels. Finally, the combination of Random Forest and Support Vector Machine (SVM) achieve the best performance. With this method, the accuracy is improved from 78% to 84% in three states classification. And in binary stress detection, the accuracy is 94%.
5. Discussion, conclusion and outlook
As presented in the previous section, 36 features are selected by Random Forest. After feature selection, the accuracy is increased from 78% to 84% in three states classification with SVM classifier. In testing, the accuracy of 94% is achieved in distinguishing only between rest and stress conditions with SVM classifier. Furthermore, in discriminating three stress levels of no, moderate and high stress conditions, the SVM classifier performs best compared to LDA, Adaboost and KNN classifier. Its accuracy is 84%. The performance of classification model is better with the combination of ECG and RSP features.
However, there is no universally accepted definition of stress. The widely used methods to assess stress are cortisol and selfreporting. But the correlations between them are only limited to 0.26–0.36 [29,30]. Furthermore, there is no standard database for stress recognition either in lab or in field. Scholars use various kinds of experiments to eliminate stress and different types of physiological data and features to recognize stress. And many physiological signals are collected using various wearable devices, such as ECG, blood pressure, electrodermal activity, photoplethysmogram. So it’s not possible to compare the accuracy among so many different studies directly.
In this work, we have got an accuracy of 84% in discriminating three stress levels. However, this work has several limitations and significant potential for future works. Firstly, we achieve this in an experimental setting. As the MIST represents a closer to real-life work stress condition, we expect similar results in real-life and long-term work experiments for field usage. Secondly, several approaches could be adopted to improve the accuracy such as better processing of data normalization, handling of physical activity confounds and combining with more physiological signals such as skin temperature and galvanic skin response. Thirdly, realtime detection and effective visualizations could be developed that permit users to visualize their stress patterns on mobile devices. This will give feedback to the users and remind them to pay attention to break. Finally, since stress may be private information for someone, we should pay more attention to privacy management for the sensor data.
In conclusion, in our study, we combine both mental and psychosocial stress factors closing to a real-life office condition. In our experiment, the monitoring of ECG and RSP signals can discriminate no, moderate and high stress levels rather than only distinguishing between rest and stress conditions. It’s more meaningful. We achieve a higher accuracy by combining Random Forest feature selection with SVM classifier. Totally we got an accuracy of 84% in discriminating three stress levels in a more comfortable way by using a wearable device.