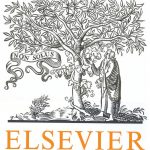
ترجمه مقاله نقش ضروری ارتباطات 6G با چشم انداز صنعت 4.0
- مبلغ: ۸۶,۰۰۰ تومان
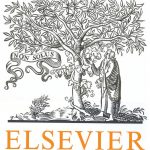
ترجمه مقاله پایداری توسعه شهری، تعدیل ساختار صنعتی و کارایی کاربری زمین
- مبلغ: ۹۱,۰۰۰ تومان
Abstract
A complex-valued Hopfield neural network (CHNN) is a multistate model of a Hopfield neural network. It has the disadvantage of low noise tolerance. Meanwhile, a symmetric CHNN (SCHNN) is a modification of a CHNN that improves noise tolerance. Furthermore, a rotor Hopfield neural network (RHNN) is an extension of a CHNN. It has twice the storage capacity of CHNNs and SCHNNs, and much better noise tolerance than CHNNs, although it requires twice many connection parameters. In this brief, we investigate the relations between CHNN, SCHNN, and RHNN; an RHNN is uniquely decomposed into a CHNN and SCHNN. In addition, the Hebbian learning rule for RHNNs is decomposed into those for CHNNs and SCHNNs.
CONCLUSION
This brief provides the unique decomposition of an RHNN into a CHNN and an SCHNN. This decomposition provides a new perspective on the RHNN, and could extend the strategy of learning algorithms. For example, it enables the corresponding CHNN and SCHNN to learn independently. The Hebbian learning rule for RHNNs is identical to the combination of those for CHNNs and SCHNNs. It is an important fact that the weighted sum input of the RHNN matches a widely linear estimation. We plan to study new learning algorithms utilizing the decomposition of RHNNs.