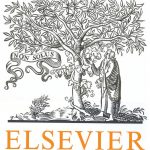
ترجمه مقاله نقش ضروری ارتباطات 6G با چشم انداز صنعت 4.0
- مبلغ: ۸۶,۰۰۰ تومان
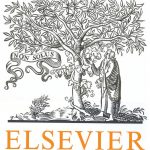
ترجمه مقاله پایداری توسعه شهری، تعدیل ساختار صنعتی و کارایی کاربری زمین
- مبلغ: ۹۱,۰۰۰ تومان
Abstract
We model the smart grid as a decentralized and hierarchical network, made up of three categories of agents: suppliers, generators and captive consumers organized in microgrids. To optimize their decisions concerning prices and traded power, agents need to forecast the demand of the microgrids and the fluctuating renewable productions. The biases resulting from the decentralized learning could create imbalances between demand and supply leading to penalties for suppliers and for generators. We analytically determine prices that provide generators with a guarantee to avoid such penalties, transferring risk to the suppliers. Additionally, we prove that collaborative learning, through coalitions of suppliers among which information is shared, minimizes the sum of their average risk. Simulations run for a large sample of parameter combinations, using external and internal regret minimization, show that the convergence of collaborative learning strategies is clearly faster than that resulting from individual learning. Finally, we analyze the suppliers’ incentives to organize in a grand coalition versus multiple coalitions, and the tightness of the learning algorithm’s theoretical bounds.
6. Conclusion
In this article, we study a model of decentralized renewable generations in which generators, suppliers and microgrids are organized into a hierarchical network. Renewable generations are modeled by random individual sequences which need not have a probabilistic structure. This general demand and supply structure is capable of taking into account exogenous events. As a result, it is more robust to extreme events and appears particularly suitable for modeling fairly erratic processes such as renewables. We analytically determine the optimal prices that enable generators to avoid the penalties that the balance operators threaten to apply when suppliers’ orders are not entirely satisfied. All the risk is then transferred to the suppliers. Additionally, we prove that the latter can minimize their average risk by sharing information in a grand coalition containing all the suppliers. Finally, numerical simulations, run on a large sample of parameter combinations, lead us to observe that the convergence times in collaborative learning, performed either through a grand coalition or through coalitions having smaller cardinality but containing more than one supplier, are clearly lower than times resulting from individual learning and that they are lower for learning algorithms based on internal regret minimization than for external regret minimization, though convergence occurs under both criteria. The tightness of convergence bound under collaborative learning is shown to be clearly better than for individual learning.