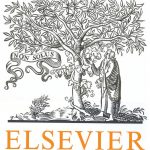
ترجمه مقاله نقش ضروری ارتباطات 6G با چشم انداز صنعت 4.0
- مبلغ: ۸۶,۰۰۰ تومان
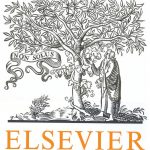
ترجمه مقاله پایداری توسعه شهری، تعدیل ساختار صنعتی و کارایی کاربری زمین
- مبلغ: ۹۱,۰۰۰ تومان
Abstract
Sentiment classification has played an important role in various research area including e-commerce applications and a number of advanced Computational Intelligence techniques including machine learning and computational linguistics have been proposed in the literature for improved sentiment classification results. While such studies focus on improving performance with new techniques or extending existing algorithms based on previously used dataset, few studies provide practitioners with insight on what techniques are better for their datasets that have different properties. This paper applies four different sentiment classification techniques from machine learning (Naïve Bayes, SVM and Decision Tree) and sentiment orientation approaches to datasets obtained from various sources (IMDB, Twitter, Hotel review, and Amazon review datasets) to learn how different data properties including dataset size, length of target documents, and subjectivity of data affect the performance of those techniques. The results of computational experiments confirm the sensitivity of the techniques on data properties including training data size, the document length and subjectivity of training /test data in the improvement of performances of techniques. The theoretical and practical implications of the findings are discussed.
6 Conclusion and future work
Sentiment classification of text data is the starting point of transforming unstructured qualitative data into quantitative data that can be used for decision making in e-commerce. Therefore sentiment classification has attracted large amount of attention from various research areas including computational intelligence, machine learning, and computational linguistics. Existing studies, however, do not paid much attention to the role of linguistic properties of datasets used in sentiment classification but instead concentrate on proposing more sophisticated and complex algorithms to improve the performance. The findings of this study suggest that researchers and practitioners need to consider the properties of datasets they have when they choose a sentiment classification algorithm.
The findings also support the contention that appropriate control of training datasets and algorithms that match to the datasets is as important as finding a sophisticated algorithm. In this regard, the study proposes practitioners and scholars with guidance on applying different sentiment classification algorithms. The study shows that the performance of classification can be improved by controlling data properties of documents in training datasets.