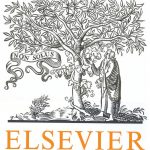
ترجمه مقاله نقش ضروری ارتباطات 6G با چشم انداز صنعت 4.0
- مبلغ: ۸۶,۰۰۰ تومان
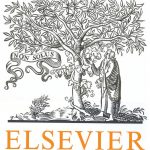
ترجمه مقاله پایداری توسعه شهری، تعدیل ساختار صنعتی و کارایی کاربری زمین
- مبلغ: ۹۱,۰۰۰ تومان
ABSTRACT
Our understanding of the spatial patterns of landslides in Africa is limited with available landslide studies typically focusing on only one or a few study areas. Moreover, Africa is clearly underrepresented in terms of available landslide inventories. This study aims to produce a first continent-wide landslide susceptibility map for Africa, calibrated with a well-distributed landslide dataset. We reviewed the literature on landslides in Africa and compiled all available landslide inventories (ca. 10,800 landslides), supplemented by additional landslide mapping using Google Earth imagery in underrepresented regions (ca. 7,250 landslides). This resulted in a dataset of approximately 18,050 landslides. Various environmental variables were investigated for their significance in explaining the observed spatial patterns of landslides. To account for potential mapping biases in the dataset, we used Monte Carlo simulations that selected different subsets of mapped landslides to test the significance of the considered environmental variables. Based on these analyses, we constructed two landslide susceptibility maps for Africa: one for all landslide types and one excluding the known rockfalls. In both maps, topography is by far the most significant variable. We evaluated the performance of the fitted multiple logistic regression models using independent subsets of landslides, selected from the total dataset. Overall, both maps perform very well in predicting intra-continental patterns of landslides in Africa and explain about 80% of the observed variance in landslide occurrence. To further test the robustness and sensitivity to mapping biases, we also modelled landslide susceptibility while excluding regions with arid climates, as landslides in these environments are expected to be better preserved over time and therefore likely relatively overrepresented. Despite this potential bias, the effect on the landslide susceptibility model is limited. Based on the constructed database and our analyses we further discuss potential research gaps for landslide prediction in Africa and at continental scales. For example, analysis of the African countries’ mean landslide susceptibility shows a lack of landslide research in various countries prone to landsliding (e.g.: Guinea, Gabon, Lesotho, Madagascar). Apart from the intrinsic value of this landslide susceptibility map as a natural hazard risk management tool, the map and compiled database are highly promising for other applications. For example, we explored the potential significance of landslides as a geomorphic process by confronting our landslide susceptibility map with an available database of measured catchment sediment yield for 500 rivers in Africa. Overall, a significant positive, but relatively weak relation between landslide susceptibility and sediment yield is observed.
4. Conclusions
In this study a first continent-wide landslide susceptibility assessment for Africa was made, based on a landslide dataset representing the entire continent. Two separate models were constructed, one including all landslide types and one excluding rockfalls that were mapped in Google Earth. Overall, both models show a very similar susceptibility pattern, largely determined by topography as the main predictor for landslide occurrence in both models. The other significant variables, i.e. seismicity, lithology and for the model excluding rockfalls also precipitation, have only a modifying effect on the overall pattern. Nevertheless, at regional scale landslide susceptibility can be easily underestimated by a factor 5, if these variables are not taken into account. Both models explain about 80% of the variance in landslide occurrence and successfully distinguish between landslide and non-landslide locations. Based on these models, about 10% of the African continent was assigned a moderate to very high landslide susceptibility. This area included 97-99% of all mapped landslides. Uncertainties on the LSS models are directly related to the predictor variables. We find the highest uncertainties for intermediate topography and areas with high precipitation and seismicity and the occurrence of siliciclastic sedimentary rocks. This points to the need for higher resolution data on these variables at the continental scale as well as the need for additional mapping efforts in such areas.