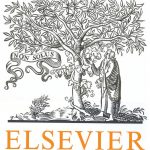
ترجمه مقاله نقش ضروری ارتباطات 6G با چشم انداز صنعت 4.0
- مبلغ: ۸۶,۰۰۰ تومان
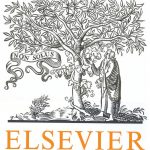
ترجمه مقاله پایداری توسعه شهری، تعدیل ساختار صنعتی و کارایی کاربری زمین
- مبلغ: ۹۱,۰۰۰ تومان
Abstract
Major advances in diagnostic technologies are offering unprecedented insight into the condition of the retina and beyond ocular disease. Digital images providing millions of morphological datasets can fast and non-invasively be analyzed in a comprehensive manner using artificial intelligence (AI). Methods based on machine learning (ML) and particularly deep learning (DL) are able to identify, localize and quantify pathological features in almost every macular and retinal disease. Convolutional neural networks thereby mimic the path of the human brain for object recognition through learning of pathological features from training sets, supervised ML, or even extrapolation from patterns recognized independently, unsupervised ML. The methods of AI-based retinal analyses are diverse and differ widely in their applicability, interpretability and reliability in different datasets and diseases. Fully automated AI-based systems have recently been approved for screening of diabetic retinopathy (DR). The overall potential of ML/DL includes screening, diagnostic grading as well as guidance of therapy with automated detection of disease activity, recurrences, quantification of therapeutic effects and identification of relevant targets for novel therapeutic approaches. Prediction and prognostic conclusions further expand the potential benefit of AI in retina which will enable personalized health care as well as large scale management and will empower the ophthalmologist to provide high quality diagnosis/therapy and successfully deal with the complexity of 21st century ophthalmology.
Discussion
The potential of AI
The digital availability of information has already vastly transformed the practice of medicine. Modern physicians use Google and PubMed more frequently than text books to aid in diagnostic and therapeutic decisions (Kluwer, 2011). This is obvious as the breadth of medical knowledge and the speed of its development grow exponentially with the interval needed to double knowledge decreasing from 3.5 years in 2010 to a predicted 0.2 years by 2020 (Densen, 2011). Patients are getting older and are affected by more and more comorbidities. Diagnostic analyses offer enormous numbers of predictors which have to be integrated into prognostic equations. It does not surprise that most diagnostic tests in medicine come back negative and misdiagnosis is common (Care et al., 2015). Leveraging dramatic advances in computational power, digital voxel matrixes underlying retinal images become thousands of individual variables. Algorithms then cluster voxels into layers and contours, reconstruct 3D features from a 2D representation and ultimately learn pathognomonic patterns and disease categories. Such digital decision support is badly needed as even the frequent grading of DR is a complex task and agreement between clinicians certified for the task and manual, but standardized, reading center gradings in DR only reached consistency in 75% (Scott et al., 2008). Introduction of an automated algorithm for DR grading compared with retinal specialist gradings achieved substantial improvements in correct adjudication, including evident DR features such as microaneurysms (Krause et al., 2018). More sophisticated but relevant features such as photoreceptor disruption is not amenable to clinical evaluation but can be identified with an accuracy, sensitivity and specificity of more than 90% using automated detection on volumetric OCT (Wang et al., 2018). In metabolic disease including diabetes, multiethnicity may play an important role requiring huge datasets for validation and evaluation, e.g., 71,896 images/494,661 images only accessible by deep learning systems, reading an AUC of 0.94 for referable and vision-threating DR (Ting et al., 2017). AI using central telemedicine systems may also support poorly resourced services in areas where human expertise is missing.