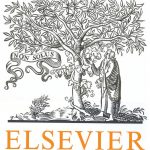
ترجمه مقاله نقش ضروری ارتباطات 6G با چشم انداز صنعت 4.0
- مبلغ: ۸۶,۰۰۰ تومان
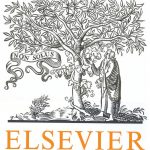
ترجمه مقاله پایداری توسعه شهری، تعدیل ساختار صنعتی و کارایی کاربری زمین
- مبلغ: ۹۱,۰۰۰ تومان
Abstract
Identifying the anomalies of wind turbine (WT) and maintaining in time will improve the reliability of wind turbine and the efficiency of energy use, however it is difficult toidentify the wind turbine’s abnormal operation by the traditional threshold settings because the anomalies can be induced by multiple factors.Therefore, this paper presents an anomaly identification model for wind turbine state parameters,and the model can identify abnormal state which the fluctuation range of the condition parametersis within the SCADA alarm threshold. The main work is as follows: 1) in order to increase the accuracy of the prediction model, a novel BPNN model integrated genetic algorithm (GA) was employed to optimize the training method (called GABP method), data samples, and input parameter selection, respectively; 2) on this basis, the distribution characteristics of state parameter prediction errors were depicted by a T-location scale (TLS) distribution with the shift factor and elastic coefficient; 3)error abnormal index (EAI) is defined to quantify the abnormal level of the prediction error, which is used as an indicator of the wind turbine anomaly. The proposed method has been applied on areal 1.5 MW wind turbine, and the analysis shows that the proposed method is effective in wind turbine anomaly identification.
Conclusions
An anomaly identification model for wind turbine state parameters was presented in this paper. The conclusion can be summarized as follows: Firstly, the wind turbine state parameter prediction model has been developed and trained by the GABP algorithm. The influence of the training algorithm, data samples and input parameters on the prediction performance of the developed models has been analyzed. 1) The GABP optimized model can provide much higher accuracy than the BPNN based model: the MSE(°C), MAE(°C), and MAPE(%) of GABP and BP with 1 minute interval time are (0.0530, 0.0367, 0.0964) and (0.0705, 0.0556, 0.1476), respectively; the MSE(°C), MAE(°C), and MAPE(%) of GABP and BP with 10 minute interval time are (0.1998, 0.1625, 0.4380) and (0.2884, 0.2255, 0.6247), respectively; the MSE(°C), MAE(°C), and MAPE(%) of GABP and BP with 15 minute interval time are (0.2701, 0.2096, 0.5435) and (0.3095, 0.2385, 0.5977), respectively. 2) The accuracy of prediction models developed by using the proposed data sampling method is higher than that trained by the current data: the MSE(°C), MAE(°C), and MAPE(%) of recent samples and samples of this paper with 1 minute interval time are (1.3822, 1.0563, 2.0231) and (1.0977, 0.6448, 1.4491), respectively; the MSE(°C), MAE(°C), and MAPE(%) of recent samples and samples of this paper with 10 minute interval time are (1.5479, 0.9888, 2.1844) and (1.2514, 0.9661, 1.8789), respectively; the MSE(°C), MAE(°C), and MAPE(%) of recent samples and samples of this paper with 15 minute interval time are (1.7643, 1.2633, 2.3431) and (1.4327, 1.1608, 2.0742), respectively. 3) Selecting the state parameters with wind speed and the parameters with large correlation as input parameters can further improve the prediction accuracy: the MSE(°C), MAE(°C), and MAPE(%) of Input parameters including a) Wind speeds and the previous monitored values, b) Ambient temperatures and the previous monitored values, c) Wind speeds, ambient temperatures and the previous monitored values, d) Wind speeds, temperature of gearbox input bearing and the previous monitored values are (1.4473, 1.0901, 2.1886), (1.2327, 0.9505, 2.0491), (1.1356, 0.8087, 1.5926), and (1.1180, 0.7692, 1.3826), respectively.