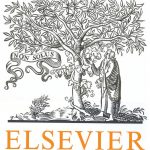
ترجمه مقاله نقش ضروری ارتباطات 6G با چشم انداز صنعت 4.0
- مبلغ: ۸۶,۰۰۰ تومان
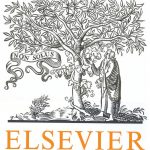
ترجمه مقاله پایداری توسعه شهری، تعدیل ساختار صنعتی و کارایی کاربری زمین
- مبلغ: ۹۱,۰۰۰ تومان
Cloud computing has emerged as a technology that grease tasks by the dynamic allocation of virtual machines. Users pay for resources based on their demand. A cloud provider has to face many challenges. One out of the essential problem is load balancing, which suffers from many issues like premature convergence, reduced convergence speed, at first chosen random solutions, and stuck in native optima. The proposed method considered the MakeSpan parameters to handle the problem related to existing met heuristic techniques. The proposed method focuses on the mutation-based Particle Swarm algorithm to balance load among the data centers. Here an efficient load balancing algorithm is developed to minimize performance parameters like MakeSpan time and improve the fitness function in cloud computing.
I. INTRODUCTION
Cloud computing has risen as jargon in the area of highperformance computing and distributed environment. It provides users with on-demand services to a shared pool of resources over the Internet which is dynamically scalable. Cloud computing is being the most magnifying field in web technologies. It dynamically allocates the task on the virtual machine which is easily accessible to the user. One of the main challenges in cloud computing is load balancing. Load balancing is a term defined for sharing the load among the multiprocessors. The workload can be classified into various categories like CPU load, network load, memory capacity issue, etc.
IV. CONCLUSION AND FUTURE WORK
Load Balancing has always been the most significant challenge in the Cloud Computing environment. The primary objective of the proposed algorithm is to minimize MakeSpan and improve fitness function. Here proposed method uses a mutation-based particle swarm optimization. It is a natureinspired optimization technique, so the proposed method combined it with a genetic operator i.e. Mutation. Applying mutation on the best solution given by the existing PSO algorithm gave us a better MakeSpan and improved the fitness function. MPSO algorithm gives better results as compared to PSO. Here proposed method is also compared on the MakeSpan parameter. The proposed method used pre-emptive virtual machine scheduling for performing load balancing. In the future, various other metrics like throughput, average time, resource utilization, waiting time, etc. can be considered.
رایانش ابری، نوعی فن آوری است که وظایف را با تخصیص دینامیکی ماشینهای مجازی اجرا میکند. هزینهای که کاربران پرداخت میکنند بر مبنای میزان تقاضای آنها برای منابع است. یک تأمین کننده ابر باید با چالشهای زیادی روبرو شود. یکی از مسائل بسیار مهم، تعادل بار است که از مشکلات زیادی مانند همگرایی نابهنگام، کاهش سرعت همگرایی، راه حلهای تصادفی که ابتدا انتخاب میشوند و درگیر شدن در اپتیمای بومی تأثیر میپذیرند. روش پیشنهادی، پارامترهای زمان صرف شده (MakeSpan) را برای رسیدگی به مسئله مربوط به تکنیکهای اکتشافی موجود مورد نظر قرار داده است. در اینجا یک الگوریتم تعادل بار کارآمد برای به حداقل رساندن پارامترهای عملکرد مانند زمان صرف شده (MakeSpan) طراحی شده که تابع برازش را در رایانش ابری بهبود میبخشد.
1. مقدمه
رایانش ابری به عنوان یک زبان حرفهای در حوزه رایانش سریع و محیط توزیع شده ایجاد شده است. این فن آوری سرویسهای درخواستی را در مجموعه مشترکی از منابع از طریق اینترنت برای کاربران فراهم میآورد که بطور پویایی مقیاس پذیر هستند. رایانش ابری یکی از بزرگترین زمینهها در فن آوریهای وب است که میتواند وظیفه ای را که کاربران نمیتوانند با راحتی به آن دسترسی داشته باشند، بطور پویایی به یک ماشین مجازی اختصاص دهد. یکی از چالشهای مهم در رایانش ابری، تعادل بار است. تعادل بار اصطلاحی است که برای تسهیم بار بین چندین پردازنده تعریف میشود. بار کاری را میتوان در دستههای مختلفی مانند بار CPU ، بار شبکه ، مشکل ظرفیت حافظه و غیره طبقه بندی کرد.
4. نتیجه گیری و کارهای آینده
تعادل بار همیشه از مهمترین چالشها در محیط رایانش ابری بوده است. هدف اصلی الگوریتم پیشنهادی، به حداقل رساندن زمان صرف شده (MakeSpan) و بهبود تابع برازش است. در اینجا روش پیشنهادی، از بهینه سازی ازدحام ذرات مبتنی بر جهش استفاده کرده است. این یک روش بهینه سازی الهام گرفته از طبیعت است، بنابراین روش پیشنهادی آن را با یک عملگر ژنتیکی یعنی جهش ترکیب کرده است. استفاده از جهش برای بهترین راه حل ارائه شده توسط الگوریتم PSO موجود ، زمان صرف شدۀ بهتری را برای ما ایجاد کرده و تابع برازش را بهبود بخشیده است. الگوریتم MPSO در مقایسه با PSO نتایج بهتری را میدهد. در اینجا روش پیشنهادی در مورد پارامتر زمان صرف شده (MakeSpan) نیز مقایسه شده است. در روش پیشنهادی ، از زمان بندی انحصاری ماشین مجازی برای اجرای تعادل بار استفاده شده است. در آینده، ، معیارهای مختلف دیگری مانند توان عملیاتی، زمان میانگین ، استفاده از منابع ، زمان انتظار و غیره را میتوان مد نظر قرار داد.