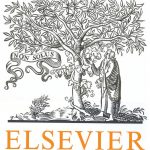
ترجمه مقاله نقش ضروری ارتباطات 6G با چشم انداز صنعت 4.0
- مبلغ: ۸۶,۰۰۰ تومان
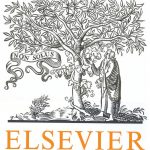
ترجمه مقاله پایداری توسعه شهری، تعدیل ساختار صنعتی و کارایی کاربری زمین
- مبلغ: ۹۱,۰۰۰ تومان
Abstract
We introduce and analyze univariate, linear, and stationary subdivision schemes for refining noisy data by fitting local least squares polynomials. This is the first attempt to design subdivision schemes for noisy data. We present primal schemes, with refinement rules based on locally fitting linear polynomials to the data, and study their convergence, smoothness, and basic limit functions. Then, we provide several numerical experiments that demonstrate the limit functions generated by these schemes from initial noisy data. The application of an advanced local linear regression method to the same data shows that the methods are comparable. In addition, several extensions and variants are discussed and their performance is illustrated by examples. We conclude by applying the schemes to noisy geometric data.
5. Application to noisy geometric data
We conclude the paper by presenting applications of our least squares subdivision schemes to noisy samples of curves and of surfaces. We measure the level of the noise by SNR, although this measure in the geometrical setting is less informative than in the functional setting, because the significance of the noise also depends highly on the geometry. 5.1. Examples of curves The parametrization of a curve enables us to apply our univariate subdivision schemes to each of its components. By doing so, we can construct an approximation to the curve from its noisy samples. We introduce two such examples. The first example consists of an alpha-like curve, given by x(t) = 3t 4 + t 2 + 1, y(t) = t 5 − 2t, (25) sampled equidistantly over [−1.4, 1.4], that is, with samples taken at ti = −7/5 + ih, where h = 14/145 and i = 0,..., 29. This curve and its sample points are shown in Figs. 14(a) and 14(b). The first set of noisy samples with a relatively low level of noise is shown in Fig. 14(c). We apply S3 and S5 (both based on linear fitting, see Section 2) to these samples, giving the limit curves in Figs. 15(a) and 15(b), respectively. The limits of both schemes retain the general shape of the curve, but a minor artifact appears on the limit curve generated by S3 since it closely fits the noisy samples. Perturbing the samples with high level noise, as seen in Fig. 14(d), reveals an overfitting by the limit curve of S3 in Fig. 15(c), while the limit curve generated by S5 in Fig. 15(d) preserves the topology of the original curve.