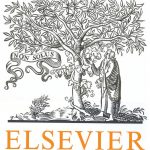
ترجمه مقاله نقش ضروری ارتباطات 6G با چشم انداز صنعت 4.0
- مبلغ: ۸۶,۰۰۰ تومان
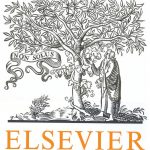
ترجمه مقاله پایداری توسعه شهری، تعدیل ساختار صنعتی و کارایی کاربری زمین
- مبلغ: ۹۱,۰۰۰ تومان
Abstract
Data Envelopment Analysis (DEA) is a nonparametric, data driven method to conduct relative performance measurements among a set of decision making units (DMUs). Efficiency scores are computed based on assessing input and output data for each DMU by means of linear programming. Traditionally, these data are assumed to be known precisely. We instead consider the situation in which data is uncertain, and in this case, we demonstrate that efficiency scores increase monotonically with uncertainty. This enables inefficient DMUs to leverage uncertainty to counter their assessment of being inefficient. Using the framework of robust optimization, we propose an uncertain DEA (uDEA) model for which an optimal solution determines 1) the maximum possible efficiency score of a DMU over all permissible uncertainties, and 2) the minimal amount of uncertainty that is required to achieve this efficiency score. We show that the uDEA model is a proper generalization of traditional DEA and provide a first-order algorithm to solve the uDEA model with ellipsoidal uncertainty sets. Finally, we present a case study applying uDEA to the problem of deciding efficiency of radiotherapy treatments.
9. Conclusion
We investigated how DEA is affected by uncertain data. We first presented a robust DEA model that defines a robust efficiency score for known uncertainty sets. We then formally showed that an increase in the uncertainty harbored by a collection of uncertainty increases the efficiency score of a DMU. This led to the question of how much uncertainty is needed to classify a DMU as efficient. We introduced the definition of an amount of uncertainty, which allowed us to formulate an optimization problem that answers this question. We then discussed configurations of uncertainty from a stochastic perspective. After illustrating our concepts with simple examples, we proved that traditional DEA is a special case of uDEA for a particular configuration of uncertainty. We also provided a first-order algorithm to solve the uDEA model with ellipsoidal uncertainty sets. Finally, we presented a case study in radiotherapy to validate the relevance of uDEA in some practical applications. We have not addressed in any detail the possible situation that configurations of uncertainty in the uDEA model depend on the DMU under assessment, which will be investigated in future work. Other questions for future research are whether and how a stochastic interpretation of uDEA opens a route to approaching the problem via simulation. The relationship between uDEA and parametric analysis will also lead to further questions.