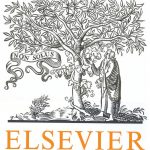
ترجمه مقاله نقش ضروری ارتباطات 6G با چشم انداز صنعت 4.0
- مبلغ: ۸۶,۰۰۰ تومان
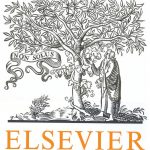
ترجمه مقاله پایداری توسعه شهری، تعدیل ساختار صنعتی و کارایی کاربری زمین
- مبلغ: ۹۱,۰۰۰ تومان
abstract
Big data analytics is the key research subject for future data driven decision making applications. Due to the large amount of data, progressive analytics could provide an efficient way for querying big data clusters. Each cluster contains only a piece of the examined data. Continuous queries over these data sources require intelligent mechanism to result the final outcome (query response) in the minimum time with the maximum performance. A Query Controller (QC) is responsible to manage continuous/sequential queries and return the final outcome to users or applications. In this paper, we propose a mechanism that can be adopted by the QC capable of managing partial results retrieved by a number of processors each one responsible for each cluster. Each processor executes a query over a specific cluster of data. The proposed mechanism adopts two sequential decision making models for handling the incoming partial results. The first model is based on a finite horizon time-optimized model and the second one is based on an infinite horizon optimally scheduled model. We provide mathematical formulations for solving the discussed problem and present simulation results. Through a large number of experiments, we reveal the advantages of the proposed models and give numerical results comparing them with a deterministic model. These results indicate that the proposed models can efficiently reduce the required time for returning the final outcome to the user/application while keeping the quality of the aggregated result at high levels.
5. Conclusions and future work
Progressive analytics can offer many advantages when adopted to manage big data. Such technique could be very efficient, especially when streams of data is the main scenario. In such cases, data are continually updated and, thus, there is not any insight on their form. In this paper, we focus on data parallelism and assume an underlying progressive analytics service. We propose a mechanism for handling responses retrieved by processors querying clusters of data. Each processor adopts a progressive analytics scheme and is responsible to return early (partial) results and a confidence value to our mechanism. We adopt the principles of the Optimal Stopping Theory (OST) and model the behaviour of a Query Controller (QC) responsible to manage multiple queries. We build on top of the processors and provide an intelligent decision making mechanism. Our aim is to alleviate users/applications from the responsibility of monitoring continuous results retrieved by processors and deciding when it is the right time to stop the process in order to save time and resources. Two models are described: the first assumes a finite horizon scheme while the second considers an infinite horizon setting. A large number of experiments reveal the efficiency of the proposed models. We focus on the throughput of the QC when working in a continuous query scenario and on the quality of the final outcome. Through our results, it is revealed that there is a trade off between throughput and the quality of the final outcome. Future extensions of our work include the definition of an intelligent scheme for creating plans and resulting assignments of queries to specific processors. Every query will be assigned to specific processors, probably, a subset of the processors available to the QC. For this, we are going to provide specific models for queries and processors characteristics. Through this approach, the efficiency of the proposed system will be maximized as the appropriate processors will be selected only for those queries that their performance will be the maximum. A learning technique will be also adopted to build an intelligent scheme for assigning queries to processors. For this, modelling the underlying data and the adoption of an algorithm that splits them to the appropriate pieces, in the most efficient way, seem to be imperative.