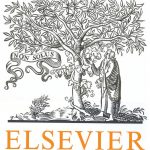
ترجمه مقاله نقش ضروری ارتباطات 6G با چشم انداز صنعت 4.0
- مبلغ: ۸۶,۰۰۰ تومان
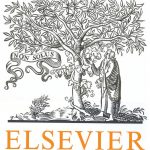
ترجمه مقاله پایداری توسعه شهری، تعدیل ساختار صنعتی و کارایی کاربری زمین
- مبلغ: ۹۱,۰۰۰ تومان
Abstract
Most of the existing studies in temporal data mining consider only lifespan of items to find general temporal association rules. However, an infrequent item for the entire time may be frequent within part of the time. We thus organize time into granules and consider temporal data mining for different levels of granules. Besides, an item may not be ready at the beginning of a store. In this paper, we use the first transaction including an item as the start point for the item. Before the start point, the item may not be brought. A three-phase mining framework with consideration of the item lifespan definition is designed. At last, experiments were made to demonstrate the performance of the proposed framework.
6. Conclusions
In this paper, we introduce a new concept of temporal association rule mining with a hierarchy of time granules to find hierarchical temporal association rules in temporal databases, and we also present the effective approach (abbreviated as TPPF) to find such rules. In particular, an effective strategy is designed to predict the upper-bound of support values for itemsets. The strategy can be used to remove unpromising itemsets at an early stage in the process, and the proposed TPPF can effectively reduce the computational cost of scanning a temporal database. Experiments were also made, with results showing the proposed TPPF outperformed the other one TP-HTAR in reducing database scan and computational time. The future research directions of this work are as follows. First, we will attempt to investigate the incremental problem of hierarchical temporal association rule mining. That is, based on this work, we will design a method to mine the new result without performing the whole mining procedure at database modification. Second, the optimal minimum support and con- fidence will be approximated by machine learning techniques. Third, actually, this work is the beginning of hierarchical temporal association rule mining. In the future, more efficient mining algorithms such as FP-growth will be adopted as the solutions to accelerate the mining process and more mining consideration such as utility mining will be studied to extend its applications.