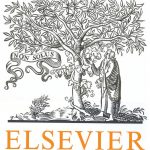
ترجمه مقاله نقش ضروری ارتباطات 6G با چشم انداز صنعت 4.0
- مبلغ: ۸۶,۰۰۰ تومان
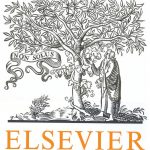
ترجمه مقاله پایداری توسعه شهری، تعدیل ساختار صنعتی و کارایی کاربری زمین
- مبلغ: ۹۱,۰۰۰ تومان
In recent years large-set parallel data processing has gained quantum as one of the predominant applications of Infrastructure-as-a-Service (IaaS) clouds. Data processing frameworks like Google's MapReduce and its open source implementation Hadoop, Microsoft's Dryad and so on are currently in use for parallel data processing in cloud-based companies. But the problem with them is that they are designed for homogeneous environments like clusters and disregard the dynamic and heterogeneous nature of a cloud. As a result, allocation and de-allocation of compute nodes at runtime is ineffective thereby increasing processing time and cost. In this paper we present our approach towards parallel data processing exploiting dynamic resource allocation in IaaS clouds. Our architecture ensures parallel data processing using Directed Acyclic task graph. To reduce the latency and to improve throughput, load balancing is introduced in the architecture. Incoming jobs are divided into tasks that are assigned to different types of virtual machines that are dynamically instantiated and terminated during job execution.