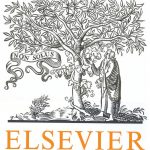
ترجمه مقاله نقش ضروری ارتباطات 6G با چشم انداز صنعت 4.0
- مبلغ: ۸۶,۰۰۰ تومان
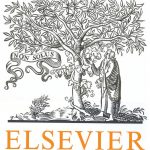
ترجمه مقاله پایداری توسعه شهری، تعدیل ساختار صنعتی و کارایی کاربری زمین
- مبلغ: ۹۱,۰۰۰ تومان
Abstract
In this paper we devise a new algorithm for completing surface with missing geometry and topology founded upon the theory and techniques of sparse signal recovery. The key intuition is that any meaningful 3D shape, represented as a discrete mesh, almost certainly possesses a low-dimensional intrinsic structure, which can be expressed as a sparse representation in some transformed domains. Instead of estimating the missing geometry directly, our novel method is to find this low-dimensional representation which describes the entire original shape. More specifically, we find that, for many shapes, the vertex coordinate function can be well approximated by a very sparse coefficient representation with respect to the dictionary comprising its Laplacian eigenfunctions, and it is then possible to recover this sparse representation from partial measurements of the original shape. Taking advantage of the sparsity cue, we advocate a novel variational approach for surface inpainting, integrating data fidelity constraints on the shape domain with coefficient sparsity constraints on the transformed domain. Because of the powerful properties of Laplacian eigenbasis, the inpainting results of our method tend to be smooth and globally coherent with the remaining shape. We demonstrate the performance of our new method via various examples in geometry restoration, shape repair, and hole filling.
6. Conclusion
In this paper, we have proposed a novel surface inpainting algorithm based on sparse signal recovery. Instead of directly estimating the local missing geometry, our new inpainting framework is designed to discover the coefficient representation of the entire original shape in a transform domain. When the shape geometry is sufficiently sparse with respect to the dictionary of transform basis, chances are we can accurately recover this sparse representation by imposing sparsity constraints on the coefficients given partial observations. In our method, we adopt the mesh Laplacian eigenbasis as dictionary, and formulate surface inpainting as a sparse signal recovery problem. Leveraging standard l1 optimization techniques, we can obtain an estimated shape which agrees with the observable parts and are globally coherent. For shapes that are highly compressible w.r.t. the Laplacian eigenbasis, we have experimentally demonstrated the great potential of our method for geometry restoration, geometry repair, and hole filling.