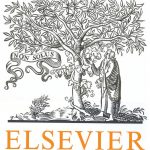
ترجمه مقاله نقش ضروری ارتباطات 6G با چشم انداز صنعت 4.0
- مبلغ: ۸۶,۰۰۰ تومان
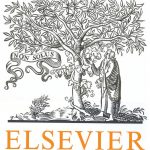
ترجمه مقاله پایداری توسعه شهری، تعدیل ساختار صنعتی و کارایی کاربری زمین
- مبلغ: ۹۱,۰۰۰ تومان
abstract
The evaluation of subjective data is a very demanding task. The classification of the information gathered from human evaluators and the possible high noise levels introduced are ones of the most difficult issues to deal with. This situation leads to adopt individuals who can be considered as experts in the specific application domain. Thus, the development of Expert Systems (ES) that consider the opinion of these individuals have been appeared to mitigate the problem. In this work an original methodology for the selection of subjective sequential data for the training of ES is presented. The system is based on the arrangement of knowledge acquired from a group of human experts. An original similarity measure between the subjective evaluations is proposed. Homogeneous groups of experts are produced using this similarity through a clustering algorithm. The methodology was applied to a practical case of the Intelligent Transportation Systems (ITS) domain for the training of ES for driving risk prediction. The results confirm the relevance of selecting homogeneous information (grouping similar opinions) when generating a ground truth (a reliable signal) for the training of ES. Further, the results show the need of considering subjective sequential data when working with phenomena where a set of rules could not be easily learned from human experts, such as risk assessment.
6. Conclusions
This paper has introduced a novel methodology for the selection of subjective sequential data for the training of ES. The methodology is based on the arrangement of homogeneous information acquired from a group of human experts. It has been proposed two different similarity measures between linearized sequential data, and a novel similarity measure that uses their combination using cluster information.
Several experiments have been achieved in order to illustrate the performance of the methodology. Three of the most representative ones have been included. The first example uses synthetic data to present the methodology. The methodology has been applied to a practical case of the ITS domain where an ES for driving risk prediction has been trained and evaluated through risk evaluations acquired from a group of traffic safety experts. An experiment has been focused on an urban scenario, and another experiment makes use of data collected from an interurban scenario.
The obtained results from these experiments have shown the relevance of selecting homogeneous information for the generation of a reliable ground truth. Also, it could be concluded that the ES trained with homogeneous evaluations performed better when predicting the driving risk. Moreover, these results show the relevance of the use of subjective sequential data when dealing with phenomena where a set of rules could not be easily acquired from human experts, such as risk assessment. In this case, the rules have been properly learned from a set of homogeneous evaluations arranged with the presented methodology obtaining outstanding results.