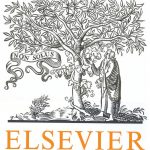
ترجمه مقاله نقش ضروری ارتباطات 6G با چشم انداز صنعت 4.0
- مبلغ: ۸۶,۰۰۰ تومان
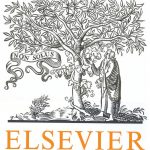
ترجمه مقاله پایداری توسعه شهری، تعدیل ساختار صنعتی و کارایی کاربری زمین
- مبلغ: ۹۱,۰۰۰ تومان
ABSTRACT
This study introduces a multistage chance-constrained stochastic model for strategic planning of battery electric vehicle (BEV) inter-city fast charging infrastructure. A mixed integer programming model is developed to determine where and when charging stations are opened, and how many chargers are required for each station to meet the growing BEV inter-city demand. The model is applied to a case study in California and solved by genetic algorithm. This study showed that investment in inter-city charging infrastructure is vital to alleviate the range anxiety. Also, planning decisions depend on many factors, such as the design level of service and vehicle range.
6. Conclusions
We successfully developed a flow-based multistage inter-city DCFC charging infrastructure expansion planning model. In response to the growing demand of BEV inter-city travels, the model integrates both an optimization model to manage facility locations of charging stations and a stochastic queuing model to determine station capacity. A genetic algorithm based heuristic method was developed to efficiently solve the problem. The model was applied to a large-scale, real-world study in California to understand the infrastructure expansion requirement in a long-term planning scope.
We found that the charging infrastructure, in terms of both location and capacity, is gradually expanded over time to meet the growing demand of BEV inter-city travels. In the baseline case, the average station size remained similar (about four chargers per station), and the most common station configuration was the single-charger station, which can provide wide spread charging infrastructure support. The actual layout strategy depends on many factors, including the actual BEV electrified range, the required level of service, and the range limitation cost. However, for most simulated scenarios, the model suggests that it is economical systems-wide to invest in inter-city DCFC charging infrastructure, even though the range limitation cost is at the low end.
One immediate extension of this study is to integrate the model with an advanced vehicle market model, such as the Market Acceptance of Advanced Automotive Technologies (MA3T) (Lin and Greene, 2010, 2011; Liu and Lin, 2017), to understand the impacts of the expanded charging infrastructure on the BEV market share. Then, a complete analysis can be conducted to evaluate inter-relationships between the BEV market share and the charging infrastructure. Another extension is to develop an efficient global optimization solution algorithm to solve this large-scale model. One immediate benefit is to be able to fully evaluate the solution quality with an attainable optimality gap. Also, this effort can help to relax the “Last-minute charging” assumption, and we can further investigate the benefits of “central decision maker” in the infrastructure requirement.