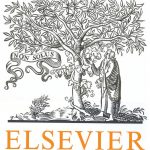
ترجمه مقاله نقش ضروری ارتباطات 6G با چشم انداز صنعت 4.0
- مبلغ: ۸۶,۰۰۰ تومان
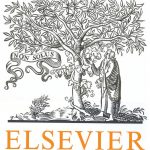
ترجمه مقاله پایداری توسعه شهری، تعدیل ساختار صنعتی و کارایی کاربری زمین
- مبلغ: ۹۱,۰۰۰ تومان
ABSTRACT
In this paper we present a stochastic model for predicting the propagation of train delays based on Bayesian networks. This method can efficiently represent and compute the complex stochastic inference between random variables. Moreover, it allows updating the probability distributions and reducing the uncertainty of future train delays in real time under the assumption that more information continuously becomes available from the monitoring system. The dynamics of a train delay over time and space is presented as a stochastic process that describes the evolution of the time-dependent random variable. This approach is further extended by modelling the interdependence between trains that share the same infrastructure or have a scheduled passenger transfer. The model is applied on a set of historical traffic realisation data from the part of a busy corridor in Sweden. We present the results and analyse the accuracy of predictions as well as the evolution of probability distributions of event delays over time. The presented method is important for making better predictions for train traffic, that are not only based on static, offline collected data, but are able to positively include the dynamic characteristics of the continuously changing delays.
Conclusions
This paper presented an analysis of train delays and their evolution in real time with respect to the dynamic stochastic phenomena. The basic idea was to characterize the effect that the prediction horizon and incoming information about running trains may have on the probability of the future train delays. Bayesian networks are proved to be an appropriate method to concisely represent the complex interdependencies between train events. The major contribution of this paper is that train delays due to interactions with other trains are adequately represented in the stochastic model. The presented method provides a way to incorporate the value of information from a live data stream into prediction of future events. A key feature for such an online learning approach is the possibility to perform good predictions under non-recurrent disruptions. That is an improvement compared to conventional prediction approaches based solely on the fixed values obtained offline from the historical data. The disruption of operation of one train causes an update of predictions for all possibly affected trains. The model was evaluated in a simulated real time environment and the computational results indicate that the predictions are reliable for horizons of up to 30 min. The practical application of this method could increase the amount of information delivered to passengers, in the form of up-todate probability for on-time arrival. It is shown by many policy studies that an informed passenger is more likely to accept this delay, and giving the probability margins could be an additional feature of projected travel time planners. Being able to characterize, analyse and predict the unavoidable dynamic uncertainty of process times can also result in better railway traffic planning and control and the corresponding tools.