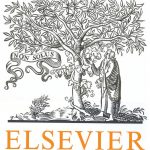
ترجمه مقاله نقش ضروری ارتباطات 6G با چشم انداز صنعت 4.0
- مبلغ: ۸۶,۰۰۰ تومان
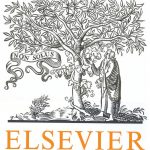
ترجمه مقاله پایداری توسعه شهری، تعدیل ساختار صنعتی و کارایی کاربری زمین
- مبلغ: ۹۱,۰۰۰ تومان
Abstract
Conventional customer relationship management (CRM) is typically based on RFM model, whose parameters are the recency, frequency and monetary aspects of target customers. The latest comprehensive analysis has enabled CRM to present parameters with time series. For example, researchers can account for changing trends based on an RFM model for flexible marketing strategies. Such changes might inspire telecommunication service scenarios that user value relies on long-term performance. In this study, we propose a statistic-based approach to value latent users via time series segmenting time interval of RFM in large scale data set. Apart from utilizing in Spark platform, we integrate multiple corresponding analysis (MCA) to regularize clustering results by the RFM model and extend these approaches to multiple levels. A comprehensive set of experiments, revealed interesting observations regarding the coexistence of time interval and RFM model. First, the clustering method along time interval in three dimensions of the RFM model outperforms the method along the three dimensions in each interval.Subsequently, the cooperation of RFM and MCA provides a convenient methodology for exploring CRM in large–scale data.Therefore, the RFM model with time intervals integrated with MCA in CRM are essential.
9. Conclusion
It is common to confront with practical input without demographical background, normally generating simple calculation and resulting in data waste. For such anonymous data, we concluded that the RFM model on Spark is a viable methodology for exploring relationships among users with the analysis of time series, while MCA is ready to prune results from the RFM model and build interaction relationships among multiple characteristics.
We propose a statistic-based approach to value latent users via time series segmenting time interval of RFM in a large–scale data set. Using time series analysis, We explored user relationships on coherent time, and we utilized the Spark platform to target users and discover quantitative relationships in the RFM model. Adjusted with k-means method, the clustering results on the three dimensions of the RFM model performed better than clustering on each interval. We leveraged MCA to correspond with multiple qualitative characteristics with quantitate results after the RFM model.
Currently, we are working on a prototype to demonstrate the changing tendency of user behavior. In the future, we will extend the time range of telecom service data to one week, to obtain a more comprehensive analysis results. Furthermore, we can conduct a prediction model and recommender model to improve our CRM analysis.