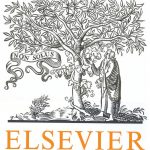
ترجمه مقاله نقش ضروری ارتباطات 6G با چشم انداز صنعت 4.0
- مبلغ: ۸۶,۰۰۰ تومان
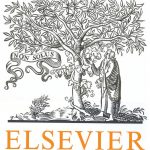
ترجمه مقاله پایداری توسعه شهری، تعدیل ساختار صنعتی و کارایی کاربری زمین
- مبلغ: ۹۱,۰۰۰ تومان
Abstract
This document presents the material of two lectures on statistical physics and neural representations, delivered by one of us (R.M.) at the Fundamental Problems in Statistical Physics XIV summer school in July 2017. In a first part, we consider the neural representations of space (maps) in the hippocampus. We introduce an extension of the Hopfield model, able to store multiple spatial maps as continuous, finite-dimensional attractors. The phase diagram and dynamical properties of the model are analyzed. We then show how spatial representations can be dynamically decoded using an effective Ising model capturing the correlation structure in the neural data, and compare applications to data obtained from hippocampal multi-electrode recordings and by (sub)sampling our attractor model. In a second part, we focus on the problem of learning data representations in machine learning, in particular with artificial neural networks. We start by introducing data representations through some illustrations. We then analyze two important algorithms, Principal Component Analysis and Restricted Boltzmann Machines, with tools from statistical physics.
6.4 Statistical mechanics of RBM
It is hopeless to provide answers to these questions in full generality for a given RBM with parameters fitted from real data. However, statistical physics methods and concepts allow us to study the typical energy landscape and properties of RBM drawn from appropriate random ensembles. We follow this approach hereafter, using the replica method [86]. We define the Random-RBM ensemble model for ReLU hidden units as follows, see also drawing in Fig. 28,
• N binary visible units, M ReLU hidden units, with N, M → ∞ and α = M N is finite.
• uniform visible layer fields, i.e. gi = g, ∀i.
• uniform hidden layer thresholds, i.e. θµ = θ, ∀µ.
• a random weight matrix wiµ = √ ξiµ N , where each ’pattern’ ξiµ is drawn independently, taking values +1, −1 with probabilities p 2 and 0 with probability 1 − p. The degree of sparsity p is the fraction of non-zero weights.