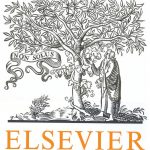
ترجمه مقاله نقش ضروری ارتباطات 6G با چشم انداز صنعت 4.0
- مبلغ: ۸۶,۰۰۰ تومان
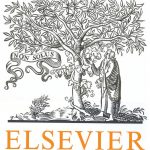
ترجمه مقاله پایداری توسعه شهری، تعدیل ساختار صنعتی و کارایی کاربری زمین
- مبلغ: ۹۱,۰۰۰ تومان
Abstract
The continuous growth of Internet and its applications caused more difficulties for analyzing Internet communications which are becoming more and more complex, this has caused new challenges for monitoring and managing the huge and vast network traffic. It is not efficient to monitor and analyze individual IP addresses, so it is more useful to monitor groups of IP addresses that have similar behavior, which represents a certain application activity. Nowadays, such a grouping is either based on network prefixes that does not meet the requirement mentioned above as difference of traffic behavior of individual IP address not being considered, or clustering IP hosts based on their traffic patterns, which requires information about TCP/UDP port numbers (which are occasionally obfuscated) or packet payloads (which are sometimes encrypted or unavailable from aggregated flow records). This paper proposes a new methodology of clustering IP addresses within a managed network domain such as campus network or ISP clients with similar social relationship based on inter-IP connectivity structure. The key idea of this methodology is to split the entire IP address space into Internal (inside the managed domain) and External (outside) ones. The clustering strategy is to group inside IP addresses that communicate with common outside IP addresses, the similarity measure of two inside IP addresses is the unique number of the common outside IP addresses. We propose a novel approach with an approximation algorithm to discover communities on a large scale in the managed domain based on the bipartite networks and one mode projection and the basis of graph partitioning of the similarity graph. Bipartite networks were built using NetFlow datasets collected from a boundary router in an actual environment, and then a one-mode projection has been applied to build a social relationship similarity graph of the inside IP addresses. We propose a community detection algorithm to extract communities. Experimental results demonstrate that our approach can discover communities from real large scale managed domain networks with a high quality. We experimentally validate our approach in terms of IP networking by applying deep flow inspection (DFI) and deep packet inspection (DPI) on related traffic to prove that hosts with the same cluster tend to have some dominant network behavior. We demonstrated the practical benefits of exploring social behavior similarity of IP hosts in understanding application usage, users’ behavior, detecting malicious users, and users of prohibited applications.
7. Conclusion
This paper presented an approach to discover IP relationship to setup a clustering model based on IP connectivity of IP addresses inside the managed domain network based on their connectivity with the outside network by observing traffic at the border router. The objective is to setup hosts’ profiles, however, since it is not efficient to setup such profiles for each IP address, it is more effi- cient to discover clusters of IP addresses with similar behaviors. Instead of clustering hosts based on their traffic patterns, this paper proposed a clustering strategy based only on the IP connectivity without any information about protocol, port, packets. Our experimental results demonstrated that this approach can discover communities from real managed domain networks. The approach is discussed and evaluated using concepts from graph partitioning, such as modularity and community detection definitions, it has been also validated by deep flow inspection DFI. To the best of our knowledge, this is the first step forward in the research to discover social communities of IP networks by splitting network into inside and outside networks and discover communities’ structure among inside network based on similarity of connectivity with the outside networks. The proposed approach is implemented in a real network, and the quality of clustering significantly fulfilled our expectations. This work has practical benefits in network security, network management, and the monitoring and analysis of large networks. The future work will include improving the algorithm for further reduction in the calculations complexity of the proposed approach.