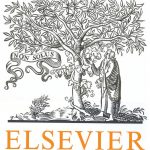
ترجمه مقاله نقش ضروری ارتباطات 6G با چشم انداز صنعت 4.0
- مبلغ: ۸۶,۰۰۰ تومان
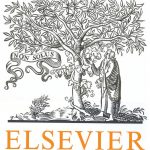
ترجمه مقاله پایداری توسعه شهری، تعدیل ساختار صنعتی و کارایی کاربری زمین
- مبلغ: ۹۱,۰۰۰ تومان
Abstract
Surrogate modelling aims to reduce computational costs by avoiding the solution of rigorous models for complex physicochemical systems. However, it requires extensive sampling to attain acceptable accuracy over the entire domain. The well-known space-filling techniques use sampling based on uniform, quasi-random, or stochastic distributions, and are typically non-adaptive. We present a novel technique to select sample points systematically in an adaptive and optimized manner, assuring that the points are placed in regions of complex behaviour and poor representation. Our proposed smart sampling algorithm (SSA) solves a series of surrogate-based nonlinear programming problems for point placement to enhance the overall accuracy and reduce computational burden. Our extensive numerical evaluations using 1-variable test problems suggest that our SSA performs the best, when its initial sample points are generated using uniform sampling. For now, this conclusion is valid for 1-variable functions only, and we are testing our algorithm for n-variable functions.
10. Conclusion
We developed a novel adaptive method (SSA) for generating the sample points required to build a surrogate approximation of a given high-fidelity function. The method begins with a set of initial sample points to generate new sample points in an iterative manner. The key novelties of our method are: (1) it combines both exploration (spatial distribution) and exploitation (surrogate quality) into a single objective for placing points, (2) it solves an optimization problem involving surrogates rather than the true function, and (3) it avoids the use of empirical scores and stochastic placement. Our extensive numerical evaluations using 1-variable test problems suggestthat our SSA performs the best, when its initial sample points are generated using uniform sampling. For now, this conclusion is valid for 1-variable functions only, and we are testing our algorithm for n-variable functions. It results in surrogates whose qualities are better than US or QS, and whose use in optimization locates the global optima quite well. A case study on styrene reactor optimization confirms the utility of our SSA for both approximation and optimization.