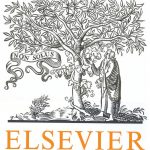
ترجمه مقاله نقش ضروری ارتباطات 6G با چشم انداز صنعت 4.0
- مبلغ: ۸۶,۰۰۰ تومان
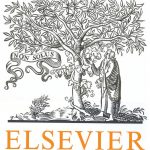
ترجمه مقاله پایداری توسعه شهری، تعدیل ساختار صنعتی و کارایی کاربری زمین
- مبلغ: ۹۱,۰۰۰ تومان
abstract
The popular volatility models focus on the conditional variance given past observations, whereas the (arguably most important) information in the current observation is ignored. This paper proposes a simple model for now-casting volatilities based on a specific ARMA representation of the log-transformed squared returns that allows us to estimate the current volatility as a function of current and past returns. The model can be viewed as a stochastic volatility model with perfect correlation between the two error terms. It is shown that the volatility nowcasts are invariant to this correlation, and therefore the estimated volatilities coincide. We propose an extension of our nowcasting model that takes into account the so-called leverage effect. The alternative models are used to estimate daily return volatilities from the S&P 500 stock price index. © 2016 International Institute of Forecasters. Published by Elsevier B.V. All rights reserved.
8. Conclusions
The proposed ARMA representation of log squared returns provides a simple method for estimating the current volatility given the past and current information on the underlying returns. Our results suggest that it outperforms the predictions of GARCH-type models and performs similarly to stochastic volatility models, while being easier to estimate. We have proposed an important extension of the model to incorporate the so-called leverage effect. Many other extensions are possible, and are indeed the object of future work. For example, it is straightforward to include a ‘‘GARCH-in-mean’’-type risk premium in the conditional mean of returns, where the risk premium depends on the current volatility, not the predicted one. Second, multivariate extensions are possible. For example, one could use a factorization as in the orthogonal GARCH model of Alexander (2001). We believe that these are important topics for future research.