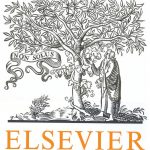
ترجمه مقاله نقش ضروری ارتباطات 6G با چشم انداز صنعت 4.0
- مبلغ: ۸۶,۰۰۰ تومان
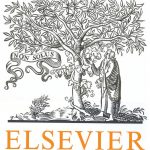
ترجمه مقاله پایداری توسعه شهری، تعدیل ساختار صنعتی و کارایی کاربری زمین
- مبلغ: ۹۱,۰۰۰ تومان
Abstract
In recent years, neural networks have enjoyed a renaissance as function approximators in reinforcement learning. Two decades after Tesauro’s TD-Gammon achieved near top-level human performance in backgammon, the deep reinforcement learning algorithm DQN achieved human-level performance in many Atari 2600 games. The purpose of this study is twofold. First, we propose two activation functions for neural network function approximation in reinforcement learning: the sigmoid-weighted linear unit (SiLU) and its derivative function (dSiLU). The activation of the SiLU is computed by the sigmoid function multiplied by its input. Second, we suggest that the more traditional approach of using on-policy learning with eligibility traces, instead of experience replay, and softmax action selection can be competitive with DQN, without the need for a separate target network. We validate our proposed approach by, first, achieving new state-of-the-art results in both stochastic SZ-Tetris and Tetris with a small 10×10 board, using TD(λ) learning and shallow dSiLU network agents, and, then, by outperforming DQN in the Atari 2600 domain by using a deep Sarsa(λ) agent with SiLU and dSiLU hidden units.
Conclusions
In this study, we proposed SiLU and dSiLU as activation functions for neural network function approximation in reinforcement learning. We demonstrated in stochastic SZ-Tetris that SiLUs significantly outperformed ReLUs, and that dSiLUs significantly outperformed sigmoid units. The best agent, the dSiLU network agent, achieved a new state-of-the-art in stochastic SZ-Tetris and in 10×10 Tetris. In the Atari 2600 domain, 365 a deep Sarsa(λ) agent with SiLUs in the convolutional layers and dSiLUs in the fullyconnected hidden layer outperformed DQN and double DQN, as measured by mean and median DQN normalized scores. An additional purpose of this study was to demonstrate that a more traditional approach of using on-policy learning with eligibility traces and softmax selection (i.e., 370 basically a “textbook” version of a reinforcement learning agent but with non-linear neural network function approximators) can be competitive with the approach used by DQN. This means that there is a lot of room for improvements, by, e.g., using, as DQN, a separate target network, but also by using more recent advances such as the dueling architecture (Wang et al., 2016) for more accurate estimates of the action values and 375 asynchronous learning by multiple agents in parallel (Mnih et al., 2016).