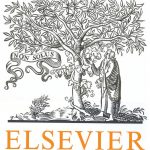
ترجمه مقاله نقش ضروری ارتباطات 6G با چشم انداز صنعت 4.0
- مبلغ: ۸۶,۰۰۰ تومان
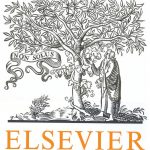
ترجمه مقاله پایداری توسعه شهری، تعدیل ساختار صنعتی و کارایی کاربری زمین
- مبلغ: ۹۱,۰۰۰ تومان
Abstract
HTTP adaptive streaming (HAS) has become the dominant technology for streaming video over the Internet. It gained popularity because of its ability to adapt the video quality to the current network conditions and other appealing properties such as usage of off-the-shelf HTTP servers and easy firewall traversal. However, when multiple HAS players share a bottleneck link for streaming, the individual adaptation techniques in the players have difficulties to maintain a stable bitrate and fairly share the network resources. HAS-assisting network elements can solve these performance problems and allow execution of advanced policies for sharing the available bandwidth. Nonetheless, testing and evaluating new sharing policies is costly and time consuming. This motivated us to formulate a model that allows to differentiate between groups of users depending on the type of user or device, and that can describe the mean bitrate of the video streams and how often this bitrate is expected to change during playout. To show how our model can be used, we demonstrate two applications of our model. Furthermore, we validate the model based results against results obtained using our streaming testbed and proxy server based HAS-assistant. The results show that our model is highly accurate for both the mean bitrate and the number of changes in video bitrate. As such, our model is a useful tool for network administrators and internet service providers for evaluating the performance of sharing policies and for managing and provisioning video delivery networks.
5. Conclusion
Video streaming over the Internet is getting extremely popular. With the rise of handheld devices such as smartphones and tablets it is no longer an exception that multiple users share a network connection for video streaming. However, when this network connection contains a bottleneck that prohibits HTTP adaptive streaming players to stream at the highest bitrates, it is important to think about how the capacity of the shared link should be shared among the players in order to provide an optimal viewing experience. Traditionally, HAS players are “selfish” in trying to achieve the highest possible video bitrate without taking into account the existence of other players in the network. With the introduction of HAS-assisting network elements, network connections can be shared more stable and fair, and policies that take into account various user and device specific factors can be executed. Developing new sharing policies for network-assisted HAS requires the policies to be thoroughly tested and evaluated. However, simulation and experimental runs are time consuming and error prone. This motivated us to formulate a Markov model that can describe the performance of network-assisted HAS under a certain policy. The model presented in this paper allows to classify different types of players or streams, and to estimate the mean bitrate and number of changes in video bitrate for each class of players. The usage of our model is demonstrated by means of a modelbased evaluation of two types of sharing policies: device heterogeneity and premium users. The model-based results are validated agains experimental runs using our streaming testbed and HASassisting proxy server. The results show to be highly accurate for both the mean bitrate and the bitrate instability. As such, our model is a useful tool that can be used in the development of sharing policies, as well as for managing and provisioning video delivery networks. Given our model, a large number of configurations can be evaluated to come to the optimal configuration given a network setting. Depending on where the bottleneck is located in the network, the model is aimed at network administrators and internet service providers. Network administrators can use the model as support while configuring HAS-assisting network elements such as our proxy server. ISPs can gain insights on HAS traffic requirements on a larger scale. Furthermore, they can use it for planning and provisioning a dedicated video-on-demand service over their IP network