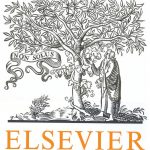
ترجمه مقاله نقش ضروری ارتباطات 6G با چشم انداز صنعت 4.0
- مبلغ: ۸۶,۰۰۰ تومان
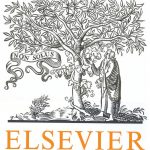
ترجمه مقاله پایداری توسعه شهری، تعدیل ساختار صنعتی و کارایی کاربری زمین
- مبلغ: ۹۱,۰۰۰ تومان
Most of the existing methods for dam behavior modeling presuppose temporal immutability of the modeled structure and require a persistent set of input parameters. In real-world applications, permanent structural changes and failures of measuring equipment can lead to a situation in which a selected model becomes unusable. Hence, the development of a system capable to automatically generate the most adequate dam model for a given situation is a necessity. In this paper, we present a self-tuning system for dam behavior modeling based on artificial neural networks (ANN) optimized for given conditions using genetic algorithms (GA). Throughout an evolutionary process, the system performs near real-time adjustment of ANN architecture according to currently active sensors and a present measurement dataset. The model was validated using the Grancarevo dam case study (at the Trebisnjica river located in the Republic of Srpska), where radial displacements of a point inside the dam structure have been modeled as a function of headwater, temperature, and ageing. The performance of the system was compared to the performance of an equivalent hybrid model based on multiple linear regression (MLR) and GA. The results of the analysis have shown that the ANN/GA hybrid can give rather better accuracy compared to the MLR/GA hybrid. On the other hand, the ANN/GA has shown higher computational demands and noticeable sensitivity to the temperature phase offset present at different geographical locations.
1. Introduction
To describe and predict the structural behavior of dams, a number of statistical, deterministic and hybrid mathematical models have been developed over the past decades. Statistical models based on multiple linear regression (MLR) and their advanced forms such as stepwise regression, robust regression, ridge regression and partial least squares regression have been shown to be more or less successful in dam modeling [1–3]. In contrast to statistical modeling, deterministic models require the solving of differential equations, for which closed form solutions could be difficult or impossible to obtain [4]. Therefore, many models that are based on numerical methods, such as the finite element method (FE), have also been developed [5]. Recently, numerical and statistical methods have been enriched with various heuristics from the artificial intelligence (AI) domain, creating hybrid models that combine their advantages.
5. Conclusion
In this paper, DEVONNA, the self-tuning software system for dam behavior modeling based on an evolving artificial neural network is presented. In order to deal with the variant set of predictor variables and the permanently increasing database of measurements in real-world problems, we developed a methodology for the generation of an ANN dam model that is optimized for given conditions using genetic algorithms. In contrast to the existing solutions, permanent variability of inputs and learning datasets require a model where most of the ANN parameters, such as the number of hidden layers, number of neurons per layer, activation function, learning algorithm and learning parameters are optimized. According to the corresponding mathematical programming model, we developed an automated system based on genetic algorithms that adapts an ANN network to fit currently active sensors and is learned using the latest historical data.
بیشتر روش های موجود برای مدلسازی رفتار سد، فرض می نماید که سازه مدل شونده به طور موقت تغییر ناپذیر است و نیاز به یک مجموعه پایدار و ثابتی از پارامتر های ورودی دارد. در کاربردهای واقعی، تغییرات دائمی سازه و خرابی های دستگاه اندازه گیری می تواند منجر به یک موقعیتی شود که در آن، یک مدل انتخاب شده، ناپایدار باشد. بنابراین، توسعه سیستمی که قادر به تولید اتوماتیک مناسب ترین مدل سد برای یک موقعیت باشد، لازم است. دراین مقاله، ما یک سیستم خود تنظیمی را برای مدلسازی رفتار سد بر مبنای شبکه های عصبی مصنوعی (ANN) بهینه شده برای شرایط مفروض با استفاده از الگوریتم های ژنتیکی (GA) ارائه می نماییم. سیستم، در کل یک فرایند تکاملی و پویا، تطبیق های تقریبا واقعی را از معماری ANN طبق سنسور های فعال جاری و یک پایگاه داده اندازه گیری موجود، اجرا می نماید. این مدل با استفاده از بررسی موردی سد Grancarvano ( در رودخانه Trebisjica در جمهوری صربستان) تایید شد جاییکه جابجایی های شعاعی یک نقطه درون سازه سد به عنوان تابعی از منبع آب جریان بالادستی (سر آب)، دما و پیرشدگی (استهلاک خواص ماده در اثر گذشت زمان)، مدل می شود. کارایی سیستم با کارایی مدل هیبریدی (دوگانه) بر مبنای رگرسیون خطی چندگانه (MLR) و GA مقایسه شد. نتایج این آنالیز نشان داده است که روش دوگانه ANN/GA می تواند دقت بهتری را در مقایسه با روش MLR/GA ارائه نماید. از سوی دیگر، روش ANN/GA، نیازمند محاسبات بیشتر بوده و حساسیت قابل توجهی را به آفست فاز دمایی موجود در مکان های جغرافیایی مختلف دارد.
1. مقدمه
برای توصیف و پیش بینی رفتار ساختاری سد، تعدادی از روش های ریاضی آماری، قطعی و دوگانه در چند دهه گذشته، توسعه داده شده است. روش های آماری بر پایه رگرسیون خطی چندگانه و شکل های پیشرفته آن مانند رگرسیون گام به گام، رگرسیون قوی، رگرسیون لبه ای و رگرسیون حداقل مربع جزئی، نشان داده شده که در مدلسازی سد کم و بیش موفق بوده اند. برعکس مدلسازی آماری، مدل های قطعی نیازمند حل معادلات دیفرانسیلی می باشد که برای آنها، بدست آوردن راه حل های شکل بسته (راه حل هایی که در تعداد محدودی از عملیات می تواند ارزیابی شود)، می تواند مشکل و یا غیر ممکن باشد. بنابراین بسیاری از مدل هایی که بر مبنای روش های عددی مانند روش المان محدود هستند، توسعه داده شده اند. اخیرا، روش های آماری و عددی با روش های حدسی و اکتشافی از حوزه هوش مصنوعی (AI) تلفیق شده که مدل های دوگانه ای را ایجاد کرده که مزیت های هر دو روش را با هم ترکیب می نماید.
5. نتیجه گیری
در این مقاله، DEVONNA، سیستم نرم افزاری خود تنظیم کننده برای مدلسازی رفتار سد بر مبنای یک شبکه عصبی مصنوعی تکاملی، ارائه شده است. به منظور کار با مجموعه متغیر از متغیر های پیش بینی کننده و افزایش دائمی پایگاه داده از اندازه گیری ها در مسائل واقعی، ما یک روشی برای تولید مدل سد ANN ارائه نمودیم که برای شرایط داده شده با استفاده از الگوریتم های ژنتیکی بهبود می یابد. برعکس راه حل های موجود، تغییر پذیری دائمی ورودی ها و پایگاه داده های یادگیری، نیاز به یک مدلی دارند که بیشتر پارامتر های ANN را مانند تعداد لایه های مخفی، تعداد نورون ها در هر لایه، تابع فعالسازی، الگوریتم یادگیری و پارامتر های یادگیری بهینه شده باشند. طبق مدل برنامه نویسی ریاضی، ما یک سیستم اتوماتیک را بر مبنای الگوریتم های ژنتیکی توسعه دادیم که یک شبکه ANN را برای تطبیق سنسور های فعالی موجود تطبیق می دهد، و با استفاده از آخرین داده تاریخچه ای، آموزش دهی می شود.