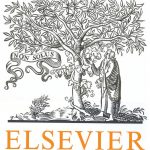
ترجمه مقاله نقش ضروری ارتباطات 6G با چشم انداز صنعت 4.0
- مبلغ: ۸۶,۰۰۰ تومان
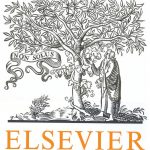
ترجمه مقاله پایداری توسعه شهری، تعدیل ساختار صنعتی و کارایی کاربری زمین
- مبلغ: ۹۱,۰۰۰ تومان
Abstract
Online social networks such as Twitter have emerged as an important mechanism for individuals to share information and post user generated content. However, filtering interesting content from the large volume of messages received through Twitter places a significant cognitive burden on users. Motivated by this problem, we develop a new automated mechanism to detect personalised interestingness, and investigate this for Twitter. Instead of undertaking semantic content analysis and matching of tweets, our approach considers the human response to content, in terms of whether the content is sufficiently stimulating to get repeatedly chosen by users for forwarding (retweeting). This approach involves machine learning against features that are relevant to a particular user and their network, to obtain an expected level of retweeting for a user and a tweet. Tweets observed to be above this expected level are classified as interesting. We implement the approach in Twitter and evaluate it using comparative human tweet assessment in two forms: through aggregated assessment using Mechanical Turk, and through a web-based experiment for Twitter users. The results provide confidence that the approach is effective in identifying the more interesting tweets from a user’s timeline. This has important implications for reduction of cognitive burden: the results show that timelines can be considerably shortened while maintaining a high degree of confidence that more interesting tweets will be retained. In conclusion we discuss how the technique could be applied to mitigate possible filter bubble effects.
5. Conclusions
In this paper we have introduced a method for scoring tweet interestingness using non-semantic methods, and we have demonstrated its ability to infer interesting tweets from the volume and noise within a user’s timeline. This has been accomplished by using comparative human tweet assessment in two forms: through aggregated assessment of interestingness using Mechanical Turk, and through a web-based experiment for individual Twitter users. The results provide confidence that the approach is effective in selecting interesting tweets from a user’s timeline. This has important implications for reduction of cognitive burden: the results show that timelines can be considerably shortened while maintaining a high degree of con- fidence that interesting tweets will be retained. The mean length of assessed timelines was 14, yet the vast majority of participants selected only one or two tweets from each as interesting, indicating that a user’s own experience of interestingness on Twitter seems less than that that might be achievable through an interestingness-based prioritisation scheme.