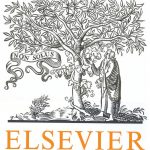
ترجمه مقاله نقش ضروری ارتباطات 6G با چشم انداز صنعت 4.0
- مبلغ: ۸۶,۰۰۰ تومان
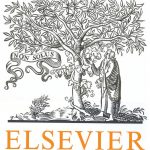
ترجمه مقاله پایداری توسعه شهری، تعدیل ساختار صنعتی و کارایی کاربری زمین
- مبلغ: ۹۱,۰۰۰ تومان
abstract
Computational offloading is the standard approach to running computationally intensive tasks on resource-limited smart devices, while reducing the local footprint, i.e., the local resource consumption. The natural candidate for computational offloading is the cloud, but recent results point out the hidden costs of cloud reliance in terms of latency and energy. Strategies that rely on local computing power have been proposed that enable fine-grained energy-aware code offloading from a mobile device to a nearby piece of infrastructure. Even state-of-the-art cloud-free solutions are centralized and suffer from a lack of flexibility, because computational offloading is tied to the presence of a specific piece of computing infrastructure. We propose AnyRun Computing (ARC), a system to dynamically select the most adequate piece of local computing infrastructure. With ARC, code can run anywhere and be offloaded not only to nearby dedicated devices, as in existing approaches, but also to peer devices. We present a detailed system description and a thorough evaluation of ARC under a wide variety of conditions. We show that ARC matches the performance of the state-of-the-art solution (MAUI), in reducing the local footprint with stationary network topology conditions and outperforms it by up to one order of magnitude under more realistic topological conditions.
7. Conclusions
We have presented ARC, a novel framework for anyrun computing, whose objective is to decide whether computational offloading to any resource-rich device willing to lend assistance is advantageous compared to local execution with respect to a rich array of performance dimensions. As changing user trends dictate an ever increasing need for computational offloading and the energy and delay footprint of cloud usage becomes well understood, we believe that anyrun offloading gets more and more attractive. While the state of the art offers solutions that presuppose the deterministic existence of higher-end computing resources, we propose a novel, flexible approach inspired by recent work in opportunistic computing whereby offloading choices are made dynamically, opportunistically, and with complete awareness of the costs and benefits involved. In this paper, we have provided a comprehensive description of our approach and we have illustrated its performance evaluation based on a custom hardware testbed for trace-based mobility emulation. Our comprehensive experimental results show that ARC proves to be extremely effective compared to MAUI [1], the stateof-the-art scheme for unirun cloud-free computational offloading. ARC virtually matches the performance of MAUI under stationary conditions and improves it by 50–60% under dynamic conditions, thus proving the potential of anyrun computational offloading.