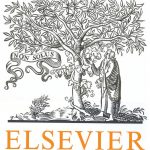
ترجمه مقاله نقش ضروری ارتباطات 6G با چشم انداز صنعت 4.0
- مبلغ: ۸۶,۰۰۰ تومان
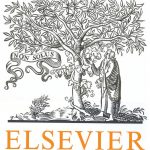
ترجمه مقاله پایداری توسعه شهری، تعدیل ساختار صنعتی و کارایی کاربری زمین
- مبلغ: ۹۱,۰۰۰ تومان
Abstract
A novel density-based clustering algorithm named QCC is presented recently. Although the algorithm has proved its strong robustness, it is still necessary to manually determine the two input parameters, including the number of neighbors (k) and the similarity threshold value (α), which severely limits the promotion of the algorithm. In addition, the QCC does not perform excellently when confronting the datasets with relatively high dimensions. To overcome these defects, firstly, we define a new method for computing local density and introduce the strategy of potential entropy into the original algorithm. Based on this idea, we propose a new QCC clustering algorithm (QCC-PE). QCC-PE can automatically extract optimal value of the parameter k by optimizing potential entropy of data field. By this means, the optimized parameter can be calculated from the datasets objectively rather than the empirical estimation accumulated from a large number of experiments. Then, t-distributed stochastic neighbor embedding (tSNE) is applied to the model of QCC-PE and further brings forward a method based on tSNE (QCC-PE-tSNE), which preprocesses high-dimensional datasets by dimensionality reduction technique. We compare the performance of the proposed algorithms with QCC, DBSCAN, and DP in the synthetic datasets, Olivetti Face Database, and real-world datasets respectively. Experimental results show that our algorithms are feasible and effective and can often outperform the comparisons.
5 Conclusions
This paper proposes a QCC-PE clustering algorithm, which focuses on the global relationship between all points on the basis of QCC and weakens the weight of k nearest neighbor in computing local density. To this end, a new method for calculating density is designed. QCC-PE can automatically determine optimal parameter k using potential entropy. Dedicated to applying to high-dimensional datasets, we incorporate the idea of dimensionality reduction which is based on t-distributed stochastic neighbor embedding and further propose QCC-PE-tSNE to improve QCC-PE. The experimental results on considerable amount of datasets demonstrate that the proposed algorithms achieve gratifying results and exhibit a promising performance advantage.