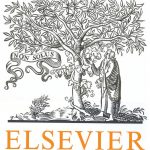
ترجمه مقاله نقش ضروری ارتباطات 6G با چشم انداز صنعت 4.0
- مبلغ: ۸۶,۰۰۰ تومان
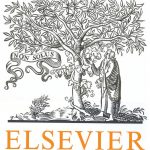
ترجمه مقاله پایداری توسعه شهری، تعدیل ساختار صنعتی و کارایی کاربری زمین
- مبلغ: ۹۱,۰۰۰ تومان
ABSTRACT
We propose the use of machine learning techniques in the Bayesian framework for the prediction of tidal currents. Computer algorithms based on the classical harmonic analysis approach have been used for several decades in tidal predictions, however the method has several limitations in terms of handling of noise, expressing uncertainty, capturing non-sinusoidal, non-harmonic variations. There is a need for principled approaches which can handle uncertainty and accommodate noise in the data. In this work, we use Gaussian processes, a Bayesian nonparametric machine learning technique, to predict tidal currents. The probabilistic and non-parametric nature of the approach enables it to represent uncertainties in modelling and deal with complexities of the problem. The method makes use of kernel functions to capture structures in the data. The overall objective is to take advantage of the recent progress in machine learning to construct a robust algorithm. Using several sets of field data, we show that the machine learning approach can achieve better results than the traditional approaches.
6. Conclusion
The problem of tidal current prediction is formulated in the framework of Bayesian GP. The goal of this work was develop robust prediction method which can address some of the shortcoming of the traditional approaches. The kernel function of the GP captures the structures in the data and amongst the wide choice of kernel functions, the periodic kernel function is in particular relevant for the present application due to its harmonic nature, without being restricted to just sinusoidal variations. The hyperparameters of the kernel functions are inferred either deterministically using MAP approach or stochastically using MCMC, with the former method being preferred for large datasets. The probabilistic nature of the machine learning approach enables it to handle uncertainty and noise, and the method generates confidence intervals directly in the time-domain (unlike HA), which would be useful in practical applications. The machine learning approach is also shown to capture nonharmonic variation using the exponentiated quadratic kernel function and can make better predictions in the short-term. We analyze and perform predictions on several sets of field data at various locations around USA. In general, the GP framework provide additional flexibilities not provided by the traditional techniques and can enhance tidal analysis. We have demonstrated that the GP based approach can achieve better accuracy than the traditional HA over a wide range of cases and varied signal characteristics. In general, enhanced prediction of currents requires better understanding of the physical processes such as the conversion of barotropic to baroclinic tides, decay of internal tides into turbulence (Egbert and Ray, 2000), meteorological effects, etc. Availability of data related to the variation of relevant parameters e.g. the meteorological forcing variables (wind stress intensity and direction) can aid in the construction of better predictive models and exploit the full potential of the machine learning algorithms.