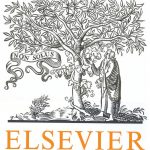
ترجمه مقاله نقش ضروری ارتباطات 6G با چشم انداز صنعت 4.0
- مبلغ: ۸۶,۰۰۰ تومان
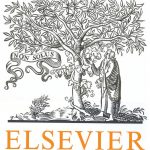
ترجمه مقاله پایداری توسعه شهری، تعدیل ساختار صنعتی و کارایی کاربری زمین
- مبلغ: ۹۱,۰۰۰ تومان
Abstract
Identification of relevant genes from microarray data is an apparent need in many applications. For such identification different ranking techniques with different evaluation criterion are used, which usually assign different ranks to the same gene. As a result, different techniques identify different gene subsets, which may not be the set of significant genes. To overcome such problems, in this study pipelining the ranking techniques is suggested. In each stage of pipeline, few of the lower ranked features are eliminated and at the end a relatively good subset of feature is preserved. However, the order in which the ranking techniques are used in the pipeline is important to ensure that the significant genes are preserved in the final subset. For this experimental study, twenty four unique pipeline models are generated out of four gene ranking strategies. These pipelines are tested with seven different microarray databases to find the suitable pipeline for such task. Further the gene subset obtained is tested with four classifiers and four performance metrics are evaluated. No single pipeline dominates other pipelines in performance; therefore a grading system is applied to the results of these pipelines to find out a consistent model. The finding of grading system that a pipeline model is significant is also established by Nemenyi post-hoc hypothetical test. Performance of this pipeline model is compared with four ranking techniques, though its performance is not superior always but majority of time it yields better results and can be suggested as a consistent model. However it requires more computational time in comparison to single ranking techniques.
5. Conclusion & future work
The contribution of proposed work is outlined as follows: • In this case study, a pipeline of gene ranking method is applied to eliminate some less significant genes at each stage ofthe pipeline. • Each of the ranking techniques used for the pipeline possesses different evaluation criterion and ranking system. Therefore very often a particular gene is assigned different rank by different ranking technique, which helps different pipelines to eliminate different set of genes at different stages and the set of genes selected after passing through different pipelines are always different. • For this work four gene ranking method with significant difference in ranking approach considered such as signal to noise ratio, pearson correlation coefficient, information gain and t-statistic to construct the pipeline. • The combination of four ranking method generates twenty four unique pipeline models, performance of which is evaluated and compared for seven publicly available gene expression databases. • Further to overrule the performance of single classifier leading to biasness, four different classifiers are used such as MLR, ANN, naïve Bayesian network and kNN. • Again the loophole of a single performance metric is taken care by considered four performance metrics for evaluation of these models. • From the simulation results it is revealed that finding a single pipeline model or group of models, suitable for best performance is difficult because performance of no single pipeline or a group dominates the rest. Therefore the technique of grading the pipeline models is employed to find out a competitive pipeline model. • To validate the effectiveness of the proposed method Nemenyi post hoc nonparametric statistical test is conducted to determine the significant difference among these models. Finally it is observed that the sequence of feature ranking techniques of the pipeline model P20 i.e. [correlation coefficient→SNR→tstatistic→information gain] is found to be the most effective in comparison to all other pipelines. • The performance of pipeline model P20 is also compared with the performance of each single ranking technique considered for construction ofthis pipeline. Itis observed that P20 does not yield the best result always in comparison to single ranking techniques, but satisfies the statistical test of significance among the single ranking techniques. Further in 63% instances P20 has obtained highest level of performance and in 38% cases it dominates the performance of all other single ranking techniques. • In majority of cases the computation time required by model P20 is much higher in comparison to all other single ranking techniques. However the application of such model is notintended for any real time system, therefore the additional computation time required can be accommodated for using a consistent model.