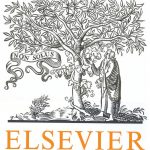
ترجمه مقاله نقش ضروری ارتباطات 6G با چشم انداز صنعت 4.0
- مبلغ: ۸۶,۰۰۰ تومان
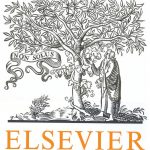
ترجمه مقاله پایداری توسعه شهری، تعدیل ساختار صنعتی و کارایی کاربری زمین
- مبلغ: ۹۱,۰۰۰ تومان
Abstract
This paper presents results of parameterisation of typical input–output relations within process flow sheet of a biodiesel plant and assesses parameterisation accuracy. A variety of scenarios were considered: 1, 2, 6 and 11 input variables (such as feed flow rate or a heater's operating temperature) were changed simultaneously, 3 domain sizes of the input variables were considered and 2 different surrogates (polynomial and high dimensional model representation (HDMR) fitting) were used. All considered outputs were heat duties of equipment within the plant. All surrogate models achieved at least a reasonable fit regardless of the domain size and number of dimensions. Global sensitivity analysis with respect to 11 inputs indicated that only 4 or fewer inputs had significant influence on any one output. Interaction terms showed only minor effects in all of the cases.
6. Conclusions
This paper presents results of parameterisation of typical inputoutput relations within process flow sheet of a biodiesel plant and assesses parameterisation accuracy. The model under investigation includes a reaction and separation steps with auxiliary equipment and was solved for steady-state operation. Thus produced data was used to generate surrogate models describing relations between chosen inputs and outputs. A variety of scenarios were considered: 1, 2, 6 and 11 input variables were changed simultaneously, 3 different domain sizes of the input variables were considered and 2 different surrogate generation methods (polynomial and HDMR fitting). Each simulation produced 400 points per input variable used for fitting and calculating R2 and R¯ 2. Test sets of points (100 points per dimension) were generated for calculating RMSD and residuals. A number of different behaviours were observed in the study. Most surrogates achieved at least a reasonable fit regardless of the domain size, number of dimensions and according to R¯ 2 and RMSD. Neither R2 nor R¯ 2 could be used to effectively differentiate between the models as most achieve values in excess of 0.98. Also, it needs to be noted that the number of parameters within HDMR fit increases far slower than within polynomial fit when high-dimensional problems are considered. The most extensive HDMR fit (H10) had far fewer parameters than polynomial fits of order >4. RMSD provides a reasonable measure for comparing accuracy of models. Fits P3 and H2b minimised RMSD and hence are the best fit for the duty of reactor 10D01 with respect to all 11 inputs. Increasing order of polynomial fit above 3 lead to poorer predictive powers due to overfitting the training data. RMSD increases exponentially for polynomial fits as the domain size of inputs increases. For fit H10 RMSD decreases from smallest to intermediate size and sharply increases from intermediate to largest size. Inclusion of 2nd order interaction terms accounted for a noticeable, but minor accuracy improvement in terms of R¯ 2 and RMSD. It was observed that nonrandomfeatures inresidualplots aremuchmoredifficultto identify when multiple inputs were considered. Higher order polynomial fits may not be suitable for describing high dimensional, chemical data. For example, performance of polynomial fit of order 5 drops from being the best model to the worst as dimensionality increases from 1 to 11.