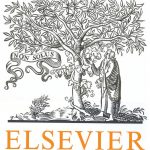
ترجمه مقاله نقش ضروری ارتباطات 6G با چشم انداز صنعت 4.0
- مبلغ: ۸۶,۰۰۰ تومان
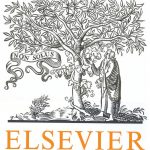
ترجمه مقاله پایداری توسعه شهری، تعدیل ساختار صنعتی و کارایی کاربری زمین
- مبلغ: ۹۱,۰۰۰ تومان
Abstract
The multi-criteria decision aid (MCDA) has been a fast growing area of operational research and management science during the past two decades. The clustering problem is one of the well-known MCDA problems, in which the K-means clustering algorithm is one of the most popular clustering algorithms. However, the existing versions of the K-means clustering algorithm are only used for partitioning the data into several clusters which don’t have priority relations. In this paper, we propose a complete ordered clustering algorithm called the ordered K-means clustering algorithm, which considers the preference degree between any two alternatives. Different from the K-means clustering algorithm, we apply the relative net flow of PROMETHEE to measure the closeness of alternatives. In this case, the ordered K-means clustering algorithm can capture the different importance degrees of criteria. At last, we employ the proposed algorithm to solve a practical ordered clustering problem concerning the human development indexes. Then a comparison analysis with an existing approach is conducted to demonstrate the advantages of the ordered K-means clustering algorithm.
5 Concluding remarks
In this paper, we have mainly considered the multi-criteria ordered clustering problems. In order to deal with this type of problems, we have proposed an ordered clustering algorithm based on K-means clustering algorithm, which is called ordered K-means clustering algorithm. As the net outranking flow is an effective way to compute the sorting of all alternatives, we have applied the idea of net outranking flow to identify the clustering center of each cluster. Different from the classical k-means clustering algorithm, the sum of all alternatives’ net outranking flow can be used as the objective function. There is a complete ordered relationship between the clusters, which is obtained from the ordered K-means clustering algorithm.
The effectiveness of the ordered K-means clustering algorithm has been illustrated by the human development index problem. The ordered clustering results obtained from the ordered K-means clustering algorithm are very highly consistent with the HDI ranks. Meanwhile, the De Smet et al.’s method [21] has been introduced to compare with the ordered K-means clustering algorithm. The comparison analysis with the De Smet et al.’s method [21] has shown that (1) the ordered K-means clustering algorithm has a good robust; (2) the ordered K-means clustering algorithm can select the suitable cluster number according to the total sum of all alternatives’ net outranking flows; (3) the clustering results obtained from the ordered K-means clustering algorithm can take full account of the intra-relationships between alternatives.
For future work, we can consider the application of the proposed OKM to other practical problems related to ordered clustering. The deep discussions of using the nonlinear preference function in the OKM are interesting. In addition, the introduction of the proposed OKM to big data clustering is also meaningful.